基准测试 XGBoost 解释
本笔记本比较了几种应用于XGBoost模型的不同解释方法。这些方法在许多不同的评估指标上进行了比较。解释误差是我们排序的主要指标,但我们也在许多其他指标上进行了比较,因为没有一个单一的指标能完全捕捉到归因解释方法的性能。
有关此处使用的每项指标的更详细解释,请查看各个类的文档字符串。
在加利福尼亚住房的XGBoost回归模型上基准测试解释器
构建模型和解释
[1]:
import warnings
import matplotlib.pyplot as plt
import numpy as np
import xgboost
from sklearn.ensemble import GradientBoostingRegressor
from sklearn.model_selection import train_test_split
import shap
import shap.benchmark
warnings.filterwarnings("ignore")
[2]:
model = GradientBoostingRegressor(subsample=0.3)
X, y = shap.datasets.california(n_points=1000)
X = X.values
X_train, X_test, y_train, y_test = train_test_split(X, y, random_state=0)
model.fit(
X_train,
y_train,
# eval_set=[(X_test, y_test)],
# early_stopping_rounds=10,
# verbose=False,
)
# define the benchmark evaluation sample set
X_eval = X_test[:]
y_eval = y_test[:]
# use an independent masker
masker = shap.maskers.Independent(X_train)
pmasker = shap.maskers.Partition(X_train)
# build the explainers
explainers = [
("Permutation", shap.PermutationExplainer(model.predict, masker)),
("Permutation part.", shap.PermutationExplainer(model.predict, pmasker)),
("Partition", shap.PartitionExplainer(model.predict, pmasker)),
("Tree", shap.TreeExplainer(model)),
("Tree approx.", shap.TreeExplainer(model, approximate=True)),
("Exact", shap.ExactExplainer(model.predict, masker)),
("Random", shap.explainers.other.Random(model.predict, masker)),
]
# # dry run to get all the code warmed up for valid runtime measurements
for name, exp in explainers:
exp(X_eval[:1])
# explain with all the explainers
attributions = [(name, exp(X_eval)) for name, exp in explainers]
运行基准测试
[3]:
results = {}
smasker = shap.benchmark.ExplanationError(masker, model.predict, X_eval)
results["explanation error"] = [smasker(v, name=n) for n, v in attributions]
ct = shap.benchmark.ComputeTime()
results["compute time"] = [ct(v, name=n) for n, v in attributions]
for mask_type, ordering in [
("keep", "positive"),
("remove", "positive"),
("keep", "negative"),
("remove", "negative"),
]:
smasker = shap.benchmark.SequentialMasker(
mask_type, ordering, masker, model.predict, X_eval
)
results[mask_type + " " + ordering] = [smasker(v, name=n) for n, v in attributions]
cmasker = shap.maskers.Composite(masker, shap.maskers.Fixed())
for mask_type, ordering in [("keep", "absolute"), ("remove", "absolute")]:
smasker = shap.benchmark.SequentialMasker(
mask_type,
ordering,
cmasker,
lambda X, y: (y - model.predict(X)) ** 2,
X_eval,
y_eval,
)
results[mask_type + " " + ordering] = [smasker(v, name=n) for n, v in attributions]
显示所有解释器在所有指标上的得分
这个多指标基准图根据第一个方法对方法进行排序,并将每个指标的分数调整为相对值,以便最佳分数出现在顶部,最差分数出现在底部。
[4]:
shap.plots.benchmark(sum(results.values(), []))
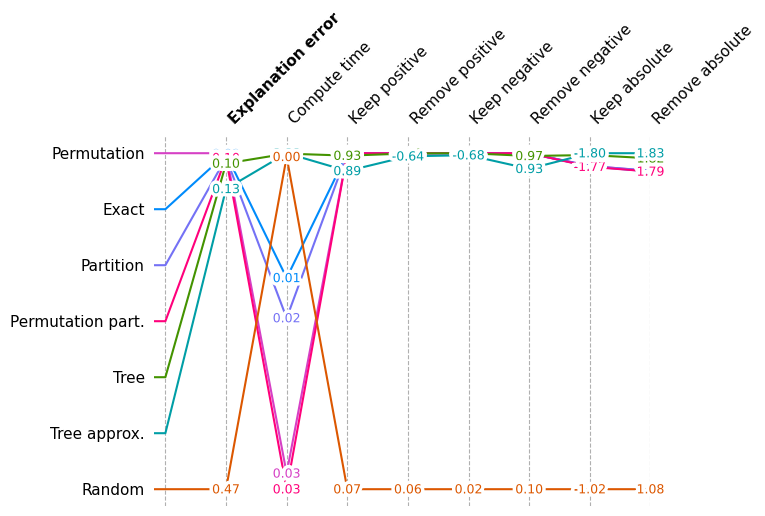
再次展示整体性能,但不包括随机因素
由于随机评分比合理的解释方法差得多,我们再次绘制相同的图表,但不包括随机方法,以便我们可以看到性能的较小变化。
[5]:
shap.plots.benchmark(filter(lambda x: x.method != "Random", sum(results.values(), [])))
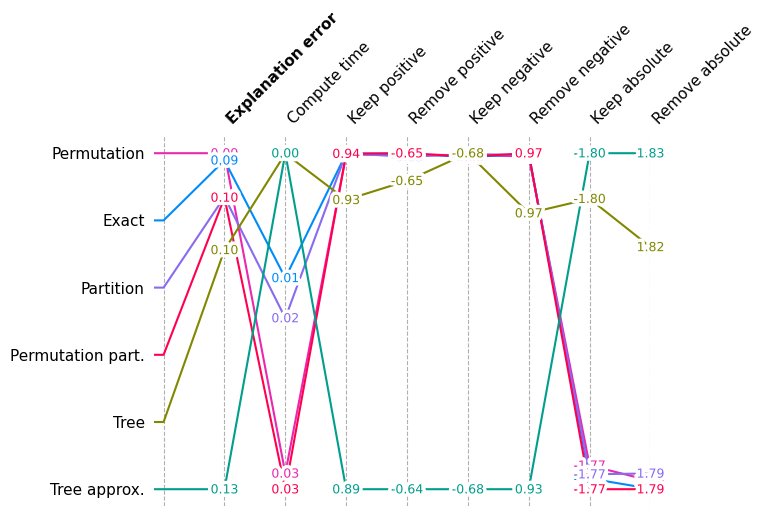
显示每种指标类型的详细图表
如果我们一次绘制一个指标的分数,那么我们可以看到方法之间更详细的比较。一些方法只有一个分数(解释误差和计算时间),而其他方法则有整个性能曲线,分数是这些曲线下的(或上的)面积。
[6]:
num_plot_rows = len(results) // 2 + len(results) % 2
fig, ax = plt.subplots(num_plot_rows, 2, figsize=(12, 5 * num_plot_rows))
for i, k in enumerate(results):
plt.subplot(num_plot_rows, 2, i + 1)
shap.plots.benchmark(results[k], show=False)
if i % 2 == 0:
ax[-1, -1].axis("off")
plt.tight_layout()
plt.show()
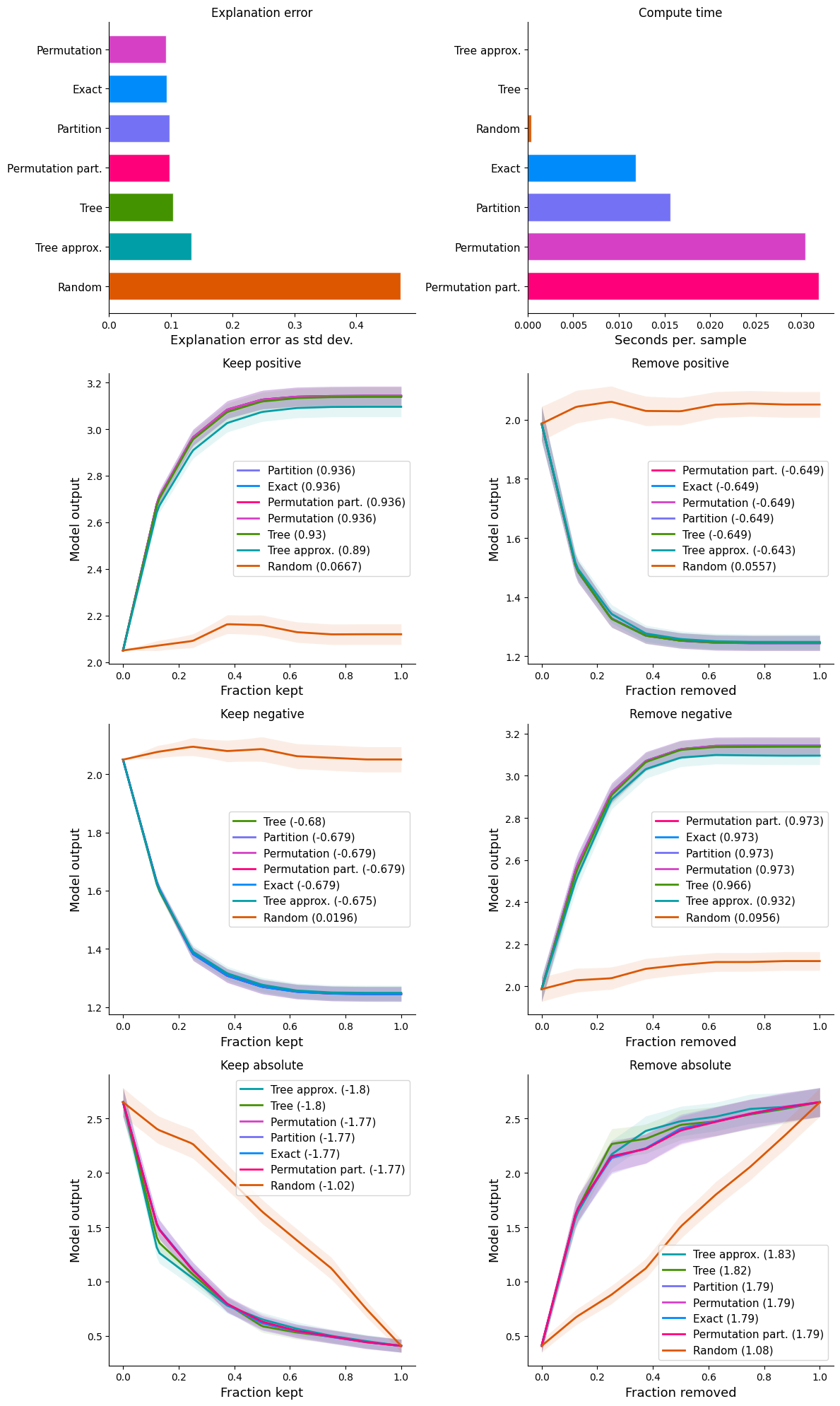
在XGBoost分类模型上对人口普查报告的收入进行解释器的基准测试
构建模型和解释
[7]:
# build the model
model = xgboost.XGBClassifier(n_estimators=1000, subsample=0.3)
X, y = shap.datasets.adult(n_points=1000)
X = X.values
X_train, X_test, y_train, y_test = train_test_split(X, y, random_state=0)
model.fit(
X_train,
y_train,
eval_set=[(X_test, y_test)],
early_stopping_rounds=10,
verbose=False,
)
def logit_predict(X):
return model.predict(X, output_margin=True)
def loss_predict(X, y):
probs = model.predict_proba(X)
return [-np.log(probs[i, y[i] * 1]) for i in range(len(y))]
# define the benchmark evaluation sample set (limited to 1000 samples for the sake of time)
X_eval = X_test[:1000]
y_eval = y_test[:1000]
# use an independent masker
masker = shap.maskers.Independent(X_train)
pmasker = shap.maskers.Partition(X_train)
# build the explainers
explainers = [
("Permutation", shap.PermutationExplainer(logit_predict, masker)),
("Permutation part.", shap.PermutationExplainer(logit_predict, pmasker)),
("Partition", shap.PartitionExplainer(logit_predict, pmasker)),
("Tree", shap.TreeExplainer(model)),
("Tree approx.", shap.TreeExplainer(model, approximate=True)),
("Random", shap.explainers.other.Random(logit_predict, masker)),
("Exact", shap.ExactExplainer(logit_predict, masker)),
]
# # dry run to get all the code warmed up for valid runtime measurements
for name, exp in explainers:
exp(X_eval[:1])
# explain with all the explainers
attributions = [(name, exp(X_eval)) for name, exp in explainers]
PartitionExplainer explainer: 251it [00:30, 5.34it/s]
运行基准测试
[8]:
results = {}
# we run explanation error first as the primary metric
smasker = shap.benchmark.ExplanationError(masker, logit_predict, X_eval)
results["explanation error"] = [smasker(v, name=n) for n, v in attributions]
# next compute time
ct = shap.benchmark.ComputeTime()
results["compute time"] = [ct(v, name=n) for n, v in attributions]
# then removal and addition of feature metrics based on model output
for mask_type, ordering in [
("keep", "positive"),
("remove", "positive"),
("keep", "negative"),
("remove", "negative"),
]:
smasker = shap.benchmark.SequentialMasker(
mask_type, ordering, masker, logit_predict, X_eval
)
results[mask_type + " " + ordering] = [smasker(v, name=n) for n, v in attributions]
# then removal and addition of feature metrics based on model loss
cmasker = shap.maskers.Composite(masker, shap.maskers.Fixed())
for mask_type, ordering in [("keep", "absolute"), ("remove", "absolute")]:
smasker = shap.benchmark.SequentialMasker(
mask_type, ordering, cmasker, loss_predict, X_eval, y_eval
)
results[mask_type + " " + ordering] = [smasker(v, name=n) for n, v in attributions]
显示所有解释器在所有指标上的总体曲线下面积得分
[9]:
shap.plots.benchmark(sum(results.values(), []))
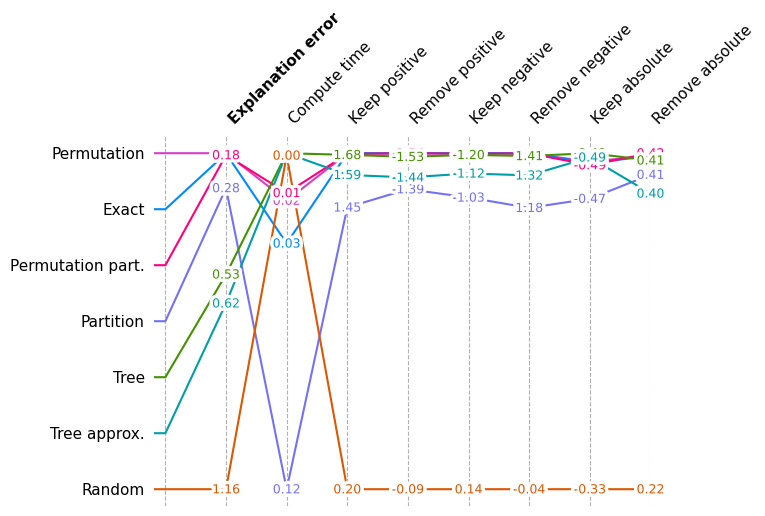
再次展示整体性能,但不包括随机因素
[10]:
shap.plots.benchmark(filter(lambda x: x.method != "Random", sum(results.values(), [])))
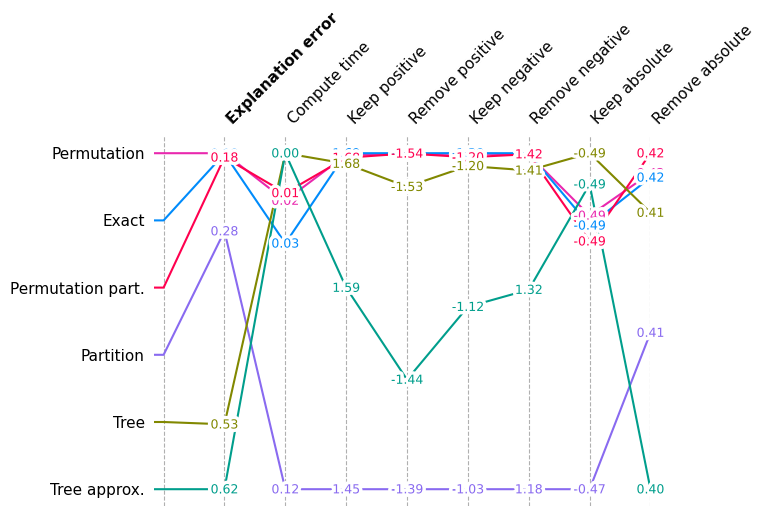
显示每种指标类型的详细图表
[11]:
num_plot_rows = len(results) // 2 + len(results) % 2
fig, ax = plt.subplots(num_plot_rows, 2, figsize=(12, 5 * num_plot_rows))
for i, k in enumerate(results):
plt.subplot(num_plot_rows, 2, i + 1)
shap.plots.benchmark(results[k], show=False)
if i % 2 == 0:
ax[-1, -1].axis("off")
plt.tight_layout()
plt.show()
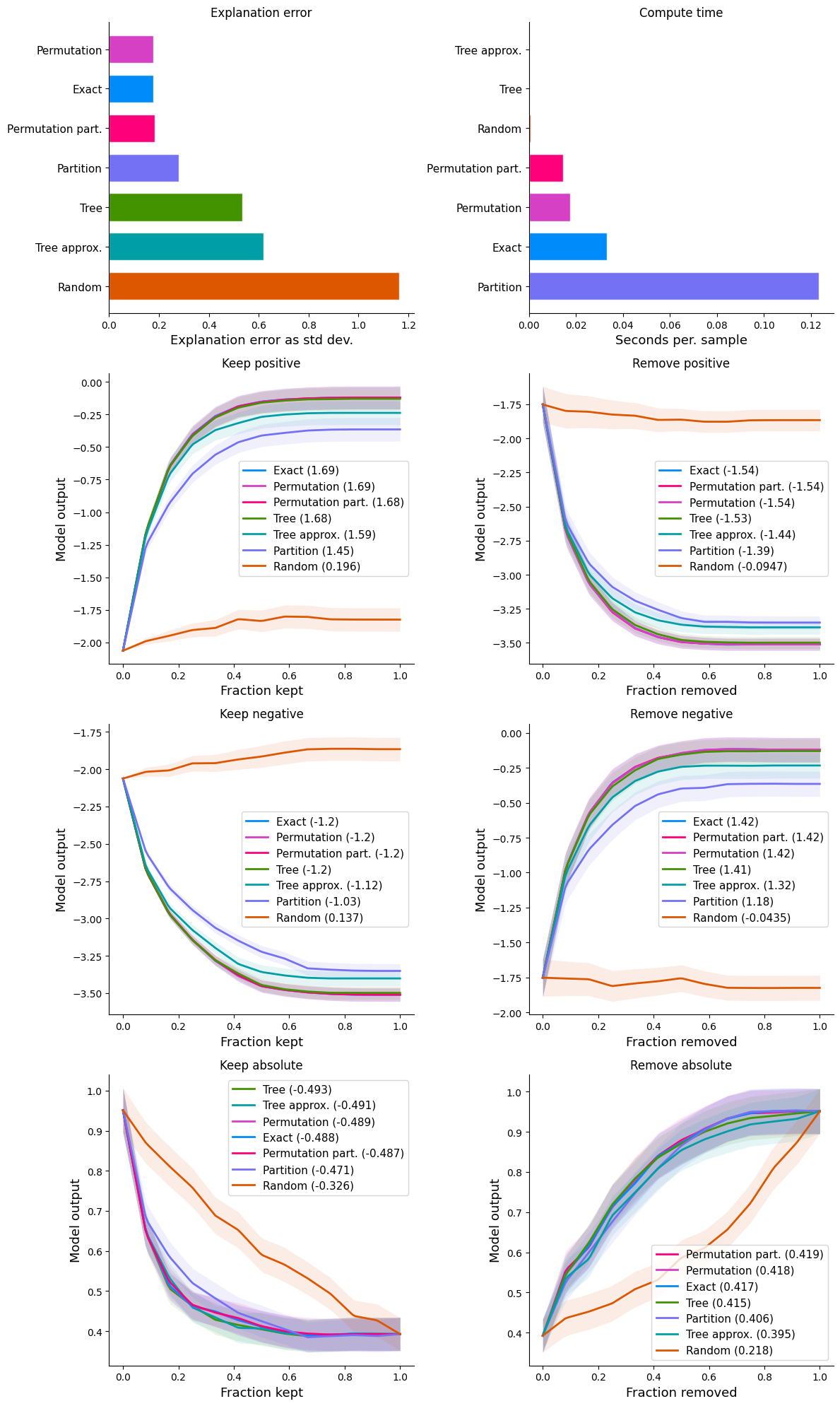
有更多有用示例的想法吗?我们鼓励提交增加此文档笔记本的拉取请求!