log_loss#
- sklearn.metrics.log_loss(y_true, y_pred, *, normalize=True, sample_weight=None, labels=None)#
Log loss,又称逻辑损失或交叉熵损失。
这是在(多项)逻辑回归及其扩展(如神经网络)中使用的损失函数,定义为对其训练数据
y_true
返回y_pred
概率的逻辑模型的负对数似然。 Log loss仅在两个或更多标签的情况下定义。 对于具有真实标签:math:y in {0,1}
和概率估计:math:p = operatorname{Pr}(y = 1)
的单个样本,log loss为:\[L_{\log}(y, p) = -(y \log (p) + (1 - y) \log (1 - p))\]在:ref:
用户指南 <log_loss>
中阅读更多内容。- Parameters:
- y_truearray-like or label indicator matrix
n_samples样本的真实(正确)标签。
- y_predarray-like of float, shape = (n_samples, n_classes) or (n_samples,)
预测概率,由分类器的predict_proba方法返回。如果
y_pred.shape = (n_samples,)
则提供的概率被认为是正类的概率。y_pred
中的标签被认为是按字母顺序排列的, 如:class:~sklearn.preprocessing.LabelBinarizer
所做。y_pred
值被剪辑到[eps, 1-eps]
,其中eps
是y_pred
数据类型的机器精度。- normalizebool, default=True
如果为true,返回每个样本的平均损失。 否则,返回每个样本损失的总和。
- sample_weightarray-like of shape (n_samples,), default=None
样本权重。
- labelsarray-like, default=None
如果未提供,标签将从y_true中推断。如果
labels
为None
且y_pred
的形状为(n_samples,),则标签被认为是二进制的,并从y_true
中推断。Added in version 0.18.
- Returns:
- lossfloat
Log loss,又称逻辑损失或交叉熵损失。
Notes
使用的对数是自然对数(以e为底)。
References
C.M. Bishop (2006). Pattern Recognition and Machine Learning. Springer, p. 209.
Examples
>>> from sklearn.metrics import log_loss >>> log_loss(["spam", "ham", "ham", "spam"], ... [[.1, .9], [.9, .1], [.8, .2], [.35, .65]]) 0.21616...
Gallery examples#
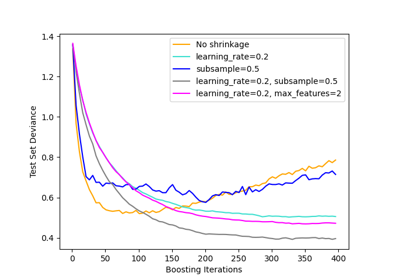
sphx_glr_auto_examples_ensemble_plot_gradient_boosting_regularization.py