使用MLflow与Tune#
MLflow 是一个开源平台,用于管理机器学习生命周期,包括实验、可重复性、部署和中央模型注册。它目前提供四个组件,包括 MLflow Tracking,以记录和查询实验,包括代码、数据、配置和结果。
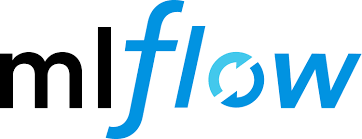
Ray Tune 当前为 MLflow Tracking 提供了两个轻量级的集成。 一个是 MLflowLoggerCallback,它会自动记录报告给 Tune 的指标到 MLflow Tracking API。
另一个是 setup_mlflow 函数,它可以与函数 API 一起使用。它会自动
使用 Tune 的训练信息初始化 MLflow API,并为每个 Tune 实验创建一个运行。
然后,在您的训练函数中,您可以像往常一样使用 MLflow,例如使用 mlflow.log_metrics()
或甚至 mlflow.autolog()
来记录您的训练过程。
运行MLflow示例#
在下面的示例中,我们将使用上述两种方法,即 MLflowLoggerCallback
和 setup_mlflow
函数来记录指标。
让我们先进行一些关键的导入:
import os
import tempfile
import time
import mlflow
from ray import train, tune
from ray.air.integrations.mlflow import MLflowLoggerCallback, setup_mlflow
接下来,我们定义一个简单的训练函数(一个Tune的Trainable
),该函数迭代地计算步骤并评估中间得分,这些得分将报告给Tune。
def evaluation_fn(step, width, height):
return (0.1 + width * step / 100) ** (-1) + height * 0.1
def train_function(config):
width, height = config["width"], config["height"]
for step in range(config.get("steps", 100)):
# 迭代训练功能 - 可以是任何任意的训练程序
intermediate_score = evaluation_fn(step, width, height)
# 将分数反馈给曲调。
train.report({"iterations": step, "mean_loss": intermediate_score})
time.sleep(0.1)
给定一个 MLFlow 跟踪 URI,您现在可以简单地将 MLflowLoggerCallback
作为 RunConfig()
中的 callback
参数使用:
def tune_with_callback(mlflow_tracking_uri, finish_fast=False):
tuner = tune.Tuner(
train_function,
tune_config=tune.TuneConfig(num_samples=5),
run_config=train.RunConfig(
name="mlflow",
callbacks=[
MLflowLoggerCallback(
tracking_uri=mlflow_tracking_uri,
experiment_name="mlflow_callback_example",
save_artifact=True,
)
],
),
param_space={
"width": tune.randint(10, 100),
"height": tune.randint(0, 100),
"steps": 5 if finish_fast else 100,
},
)
results = tuner.fit()
要使用 setup_mlflow
工具,只需在训练函数中调用此函数。 请注意,我们还使用 mlflow.log_metrics(...)
将指标记录到 MLflow。 否则,这个版本的训练函数与其原始版本相同。
def train_function_mlflow(config):
tracking_uri = config.pop("tracking_uri", None)
setup_mlflow(
config,
experiment_name="setup_mlflow_example",
tracking_uri=tracking_uri,
)
# 超参数
width, height = config["width"], config["height"]
for step in range(config.get("steps", 100)):
# 迭代训练功能 - 可以是任何任意的训练程序
intermediate_score = evaluation_fn(step, width, height)
# 将指标记录到mlflow
mlflow.log_metrics(dict(mean_loss=intermediate_score), step=step)
# 将分数反馈给曲调。
train.report({"iterations": step, "mean_loss": intermediate_score})
time.sleep(0.1)
准备好这个新的目标函数后,您现在可以按如下方式创建一个 Tune 运行:
def tune_with_setup(mlflow_tracking_uri, finish_fast=False):
# 设置实验,如果不存在则创建一个新实验。
mlflow.set_tracking_uri(mlflow_tracking_uri)
mlflow.set_experiment(experiment_name="setup_mlflow_example")
tuner = tune.Tuner(
train_function_mlflow,
tune_config=tune.TuneConfig(num_samples=5),
run_config=train.RunConfig(
name="mlflow",
),
param_space={
"width": tune.randint(10, 100),
"height": tune.randint(0, 100),
"steps": 5 if finish_fast else 100,
"tracking_uri": mlflow.get_tracking_uri(),
},
)
results = tuner.fit()
如果您恰好拥有一个 MLFlow 跟踪 URI,您可以在 mlflow_tracking_uri
变量中设置它,并将 smoke_test=False
。
否则,您可以直接运行 tune_function
和 tune_decorated
函数的快速测试,而无需使用 MLflow。
smoke_test = True
if smoke_test:
mlflow_tracking_uri = os.path.join(tempfile.gettempdir(), "mlruns")
else:
mlflow_tracking_uri = "<MLFLOW_TRACKING_URI>"
tune_with_callback(mlflow_tracking_uri, finish_fast=smoke_test)
if not smoke_test:
df = mlflow.search_runs(
[mlflow.get_experiment_by_name("mlflow_callback_example").experiment_id]
)
print(df)
tune_with_setup(mlflow_tracking_uri, finish_fast=smoke_test)
if not smoke_test:
df = mlflow.search_runs(
[mlflow.get_experiment_by_name("setup_mlflow_example").experiment_id]
)
print(df)
2022-12-22 10:37:53,580 INFO worker.py:1542 -- Started a local Ray instance. View the dashboard at http://127.0.0.1:8265
Tune Status
Current time: | 2022-12-22 10:38:04 |
Running for: | 00:00:06.73 |
Memory: | 10.4/16.0 GiB |
System Info
Using FIFO scheduling algorithm.Resources requested: 0/16 CPUs, 0/0 GPUs, 0.0/4.03 GiB heap, 0.0/2.0 GiB objects
Trial Status
Trial name | status | loc | height | width | loss | iter | total time (s) | iterations | neg_mean_loss |
---|---|---|---|---|---|---|---|---|---|
train_function_b275b_00000 | TERMINATED | 127.0.0.1:801 | 66 | 36 | 7.24935 | 5 | 0.587302 | 4 | -7.24935 |
train_function_b275b_00001 | TERMINATED | 127.0.0.1:813 | 33 | 35 | 3.96667 | 5 | 0.507423 | 4 | -3.96667 |
train_function_b275b_00002 | TERMINATED | 127.0.0.1:814 | 75 | 29 | 8.29365 | 5 | 0.518995 | 4 | -8.29365 |
train_function_b275b_00003 | TERMINATED | 127.0.0.1:815 | 28 | 63 | 3.18168 | 5 | 0.567739 | 4 | -3.18168 |
train_function_b275b_00004 | TERMINATED | 127.0.0.1:816 | 20 | 18 | 3.21951 | 5 | 0.526536 | 4 | -3.21951 |
Trial Progress
Trial name | date | done | episodes_total | experiment_id | experiment_tag | hostname | iterations | iterations_since_restore | mean_loss | neg_mean_loss | node_ip | pid | time_since_restore | time_this_iter_s | time_total_s | timestamp | timesteps_since_restore | timesteps_total | training_iteration | trial_id | warmup_time |
---|---|---|---|---|---|---|---|---|---|---|---|---|---|---|---|---|---|---|---|---|---|
train_function_b275b_00000 | 2022-12-22_10-38-01 | True | 28feaa4dd8ab4edab810e8109e77502e | 0_height=66,width=36 | kais-macbook-pro.anyscale.com.beta.tailscale.net | 4 | 5 | 7.24935 | -7.24935 | 127.0.0.1 | 801 | 0.587302 | 0.126818 | 0.587302 | 1671705481 | 0 | 5 | b275b_00000 | 0.00293493 | ||
train_function_b275b_00001 | 2022-12-22_10-38-04 | True | 245010d0c3d0439ebfb664764ae9db3c | 1_height=33,width=35 | kais-macbook-pro.anyscale.com.beta.tailscale.net | 4 | 5 | 3.96667 | -3.96667 | 127.0.0.1 | 813 | 0.507423 | 0.122086 | 0.507423 | 1671705484 | 0 | 5 | b275b_00001 | 0.00553799 | ||
train_function_b275b_00002 | 2022-12-22_10-38-04 | True | 898afbf9b906448c980f399c72a2324c | 2_height=75,width=29 | kais-macbook-pro.anyscale.com.beta.tailscale.net | 4 | 5 | 8.29365 | -8.29365 | 127.0.0.1 | 814 | 0.518995 | 0.123554 | 0.518995 | 1671705484 | 0 | 5 | b275b_00002 | 0.0040431 | ||
train_function_b275b_00003 | 2022-12-22_10-38-04 | True | 03a4476f82734642b6ab0a5040ca58f8 | 3_height=28,width=63 | kais-macbook-pro.anyscale.com.beta.tailscale.net | 4 | 5 | 3.18168 | -3.18168 | 127.0.0.1 | 815 | 0.567739 | 0.125471 | 0.567739 | 1671705484 | 0 | 5 | b275b_00003 | 0.00406194 | ||
train_function_b275b_00004 | 2022-12-22_10-38-04 | True | ff8c7c55ce6e404f9b0552c17f7a0c40 | 4_height=20,width=18 | kais-macbook-pro.anyscale.com.beta.tailscale.net | 4 | 5 | 3.21951 | -3.21951 | 127.0.0.1 | 816 | 0.526536 | 0.123327 | 0.526536 | 1671705484 | 0 | 5 | b275b_00004 | 0.00332022 |
2022-12-22 10:38:04,477 INFO tune.py:772 -- Total run time: 7.99 seconds (6.71 seconds for the tuning loop).
Tune Status
Current time: | 2022-12-22 10:38:11 |
Running for: | 00:00:07.00 |
Memory: | 10.7/16.0 GiB |
System Info
Using FIFO scheduling algorithm.Resources requested: 0/16 CPUs, 0/0 GPUs, 0.0/4.03 GiB heap, 0.0/2.0 GiB objects
Trial Status
Trial name | status | loc | height | width | loss | iter | total time (s) | iterations | neg_mean_loss |
---|---|---|---|---|---|---|---|---|---|
train_function_mlflow_b73bd_00000 | TERMINATED | 127.0.0.1:842 | 37 | 68 | 4.05461 | 5 | 0.750435 | 4 | -4.05461 |
train_function_mlflow_b73bd_00001 | TERMINATED | 127.0.0.1:853 | 50 | 20 | 6.11111 | 5 | 0.652748 | 4 | -6.11111 |
train_function_mlflow_b73bd_00002 | TERMINATED | 127.0.0.1:854 | 38 | 83 | 4.0924 | 5 | 0.6513 | 4 | -4.0924 |
train_function_mlflow_b73bd_00003 | TERMINATED | 127.0.0.1:855 | 15 | 93 | 1.76178 | 5 | 0.650586 | 4 | -1.76178 |
train_function_mlflow_b73bd_00004 | TERMINATED | 127.0.0.1:856 | 75 | 43 | 8.04945 | 5 | 0.656046 | 4 | -8.04945 |
Trial Progress
Trial name | date | done | episodes_total | experiment_id | experiment_tag | hostname | iterations | iterations_since_restore | mean_loss | neg_mean_loss | node_ip | pid | time_since_restore | time_this_iter_s | time_total_s | timestamp | timesteps_since_restore | timesteps_total | training_iteration | trial_id | warmup_time |
---|---|---|---|---|---|---|---|---|---|---|---|---|---|---|---|---|---|---|---|---|---|
train_function_mlflow_b73bd_00000 | 2022-12-22_10-38-08 | True | 62703cfe82e54d74972377fbb525b000 | 0_height=37,width=68 | kais-macbook-pro.anyscale.com.beta.tailscale.net | 4 | 5 | 4.05461 | -4.05461 | 127.0.0.1 | 842 | 0.750435 | 0.108625 | 0.750435 | 1671705488 | 0 | 5 | b73bd_00000 | 0.0030272 | ||
train_function_mlflow_b73bd_00001 | 2022-12-22_10-38-11 | True | 03ea89852115465392ed318db8021614 | 1_height=50,width=20 | kais-macbook-pro.anyscale.com.beta.tailscale.net | 4 | 5 | 6.11111 | -6.11111 | 127.0.0.1 | 853 | 0.652748 | 0.110796 | 0.652748 | 1671705491 | 0 | 5 | b73bd_00001 | 0.00303078 | ||
train_function_mlflow_b73bd_00002 | 2022-12-22_10-38-11 | True | 3731fc2966f9453ba58c650d89035ab4 | 2_height=38,width=83 | kais-macbook-pro.anyscale.com.beta.tailscale.net | 4 | 5 | 4.0924 | -4.0924 | 127.0.0.1 | 854 | 0.6513 | 0.108578 | 0.6513 | 1671705491 | 0 | 5 | b73bd_00002 | 0.00310016 | ||
train_function_mlflow_b73bd_00003 | 2022-12-22_10-38-11 | True | fb35841742b348b9912d10203c730f1e | 3_height=15,width=93 | kais-macbook-pro.anyscale.com.beta.tailscale.net | 4 | 5 | 1.76178 | -1.76178 | 127.0.0.1 | 855 | 0.650586 | 0.109097 | 0.650586 | 1671705491 | 0 | 5 | b73bd_00003 | 0.0576491 | ||
train_function_mlflow_b73bd_00004 | 2022-12-22_10-38-11 | True | 6d3cbf9ecc3446369e607ff78c67bc29 | 4_height=75,width=43 | kais-macbook-pro.anyscale.com.beta.tailscale.net | 4 | 5 | 8.04945 | -8.04945 | 127.0.0.1 | 856 | 0.656046 | 0.109869 | 0.656046 | 1671705491 | 0 | 5 | b73bd_00004 | 0.00265694 |
2022-12-22 10:38:11,514 INFO tune.py:772 -- Total run time: 7.01 seconds (6.98 seconds for the tuning loop).
这完成了我们的 Tune 和 MLflow 的操作演示。 在接下来的部分中,您可以找到有关 Tune-MLflow 集成 API 的更多详细信息。
MLflow 自动登录#
您还可以查看 here,了解如何利用 MLflow 自动登录,在本例中使用 Pytorch Lightning。
MLflow 日志记录器 API#
- class ray.air.integrations.mlflow.MLflowLoggerCallback(tracking_uri: str | None = None, *, registry_uri: str | None = None, experiment_name: str | None = None, tags: Dict | None = None, tracking_token: str | None = None, save_artifact: bool = False)[源代码]
MLflow Logger to automatically log Tune results and config to MLflow.
MLflow (https://mlflow.org) Tracking is an open source library for recording and querying experiments. This Ray Tune
LoggerCallback
sends information (config parameters, training results & metrics, and artifacts) to MLflow for automatic experiment tracking.Keep in mind that the callback will open an MLflow session on the driver and not on the trainable. Therefore, it is not possible to call MLflow functions like
mlflow.log_figure()
inside the trainable as there is no MLflow session on the trainable. For more fine grained control, useray.air.integrations.mlflow.setup_mlflow()
.- 参数:
tracking_uri – The tracking URI for where to manage experiments and runs. This can either be a local file path or a remote server. This arg gets passed directly to mlflow initialization. When using Tune in a multi-node setting, make sure to set this to a remote server and not a local file path.
registry_uri – The registry URI that gets passed directly to mlflow initialization.
experiment_name – The experiment name to use for this Tune run. If the experiment with the name already exists with MLflow, it will be reused. If not, a new experiment will be created with that name.
tags – An optional dictionary of string keys and values to set as tags on the run
tracking_token – Tracking token used to authenticate with MLflow.
save_artifact – If set to True, automatically save the entire contents of the Tune local_dir as an artifact to the corresponding run in MlFlow.
Example:
from ray.air.integrations.mlflow import MLflowLoggerCallback tags = { "user_name" : "John", "git_commit_hash" : "abc123"} tune.run( train_fn, config={ # define search space here "parameter_1": tune.choice([1, 2, 3]), "parameter_2": tune.choice([4, 5, 6]), }, callbacks=[MLflowLoggerCallback( experiment_name="experiment1", tags=tags, save_artifact=True)])
MLflow 设置 API#
- ray.air.integrations.mlflow.setup_mlflow(config: Dict | None = None, tracking_uri: str | None = None, registry_uri: str | None = None, experiment_id: str | None = None, experiment_name: str | None = None, tracking_token: str | None = None, artifact_location: str | None = None, run_name: str | None = None, create_experiment_if_not_exists: bool = False, tags: Dict | None = None, rank_zero_only: bool = True) ModuleType | _NoopModule [源代码]
Set up a MLflow session.
This function can be used to initialize an MLflow session in a (distributed) training or tuning run. The session will be created on the trainable.
By default, the MLflow experiment ID is the Ray trial ID and the MLlflow experiment name is the Ray trial name. These settings can be overwritten by passing the respective keyword arguments.
The
config
dict is automatically logged as the run parameters (excluding the mlflow settings).In distributed training with Ray Train, only the zero-rank worker will initialize mlflow. All other workers will return a noop client, so that logging is not duplicated in a distributed run. This can be disabled by passing
rank_zero_only=False
, which will then initialize mlflow in every training worker.This function will return the
mlflow
module or a noop module for non-rank zero workersif rank_zero_only=True
. By usingmlflow = setup_mlflow(config)
you can ensure that only the rank zero worker calls the mlflow API.- 参数:
config – Configuration dict to be logged to mlflow as parameters.
tracking_uri – The tracking URI for MLflow tracking. If using Tune in a multi-node setting, make sure to use a remote server for tracking.
registry_uri – The registry URI for the MLflow model registry.
experiment_id – The id of an already created MLflow experiment. All logs from all trials in
tune.Tuner()
will be reported to this experiment. If this is not provided or the experiment with this id does not exist, you must provide an``experiment_name``. This parameter takes precedence overexperiment_name
.experiment_name – The name of an already existing MLflow experiment. All logs from all trials in
tune.Tuner()
will be reported to this experiment. If this is not provided, you must provide a validexperiment_id
.tracking_token – A token to use for HTTP authentication when logging to a remote tracking server. This is useful when you want to log to a Databricks server, for example. This value will be used to set the MLFLOW_TRACKING_TOKEN environment variable on all the remote training processes.
artifact_location – The location to store run artifacts. If not provided, MLFlow picks an appropriate default. Ignored if experiment already exists.
run_name – Name of the new MLflow run that will be created. If not set, will default to the
experiment_name
.create_experiment_if_not_exists – Whether to create an experiment with the provided name if it does not already exist. Defaults to False.
tags – Tags to set for the new run.
rank_zero_only – If True, will return an initialized session only for the rank 0 worker in distributed training. If False, will initialize a session for all workers. Defaults to True.
示例
Per default, you can just call
setup_mlflow
and continue to use MLflow like you would normally do:from ray.air.integrations.mlflow import setup_mlflow def training_loop(config): mlflow = setup_mlflow(config) # ... mlflow.log_metric(key="loss", val=0.123, step=0)
In distributed data parallel training, you can utilize the return value of
setup_mlflow
. This will make sure it is only invoked on the first worker in distributed training runs.from ray.air.integrations.mlflow import setup_mlflow def training_loop(config): mlflow = setup_mlflow(config) # ... mlflow.log_metric(key="loss", val=0.123, step=0)
You can also use MlFlow’s autologging feature if using a training framework like Pytorch Lightning, XGBoost, etc. More information can be found here (https://mlflow.org/docs/latest/tracking.html#automatic-logging).
from ray.air.integrations.mlflow import setup_mlflow def train_fn(config): mlflow = setup_mlflow(config) mlflow.autolog() xgboost_results = xgb.train(config, ...)
PublicAPI (alpha): This API is in alpha and may change before becoming stable.
更多 MLflow 示例#
MLflow PyTorch Lightning 示例:使用 MLflow 和 Pytorch Lightning 结合 Ray Tune 的示例。