备注
转到末尾 下载完整示例代码。或在您的浏览器中通过 Binder 运行此示例。
保留纹理的非局部均值去噪#
在这个例子中,我们使用非局部均值滤波器对宇航员图像的细节进行去噪。非局部均值算法通过选择其他像素值的平均值来替换一个像素的值:以其他像素为中心的小块与以感兴趣像素为中心的块进行比较,并且仅对那些与当前块接近的像素进行平均。因此,该算法可以很好地恢复纹理,而这些纹理可能会被其他去噪算法模糊。
当 fast_mode
参数为 False
时,在计算补丁距离时会应用空间高斯加权。当 fast_mode
为 True
时,会应用一种更快的算法,该算法对补丁采用均匀的空间加权。
对于这两种情况,如果提供了噪声标准差 sigma
,则在计算补丁距离时会减去预期的噪声方差。这可以略微提高图像质量。
estimate_sigma
函数可以为非局部均值算法的 h``(以及可选的 ``sigma
)参数设置提供一个良好的起点。h
是一个常数,用于控制补丁权重随补丁间距离的衰减。较大的 h
允许在不同补丁之间进行更多的平滑处理。
在这个演示中,h
是手动调整以达到每个变体的近似最佳性能。
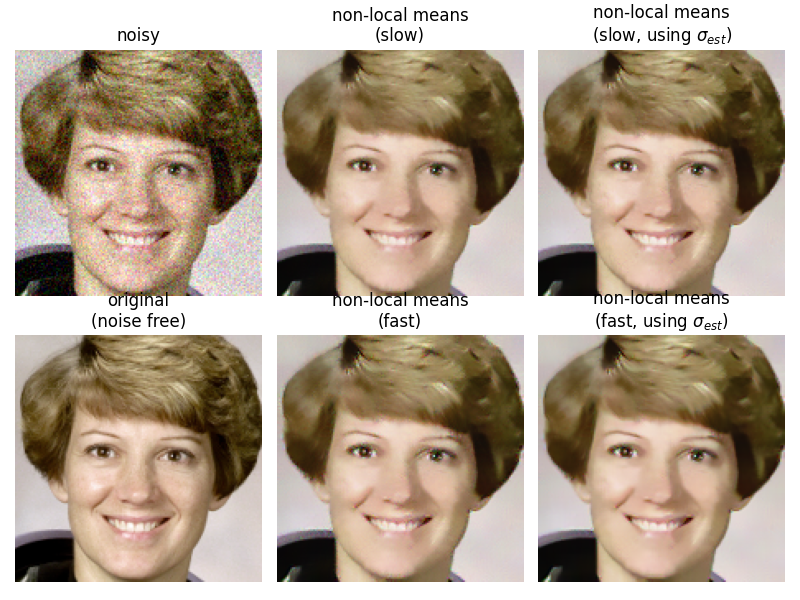
estimated noise standard deviation = 0.07842018359389026
PSNR (noisy) = 22.22
PSNR (slow) = 29.43
PSNR (slow, using sigma) = 29.84
PSNR (fast) = 29.00
PSNR (fast, using sigma) = 29.37
import numpy as np
import matplotlib.pyplot as plt
from skimage import data, img_as_float
from skimage.restoration import denoise_nl_means, estimate_sigma
from skimage.metrics import peak_signal_noise_ratio
from skimage.util import random_noise
astro = img_as_float(data.astronaut())
astro = astro[30:180, 150:300]
sigma = 0.08
noisy = random_noise(astro, var=sigma**2)
# estimate the noise standard deviation from the noisy image
sigma_est = np.mean(estimate_sigma(noisy, channel_axis=-1))
print(f'estimated noise standard deviation = {sigma_est}')
patch_kw = dict(
patch_size=5, patch_distance=6, channel_axis=-1 # 5x5 patches # 13x13 search area
)
# slow algorithm
denoise = denoise_nl_means(noisy, h=1.15 * sigma_est, fast_mode=False, **patch_kw)
# slow algorithm, sigma provided
denoise2 = denoise_nl_means(
noisy, h=0.8 * sigma_est, sigma=sigma_est, fast_mode=False, **patch_kw
)
# fast algorithm
denoise_fast = denoise_nl_means(noisy, h=0.8 * sigma_est, fast_mode=True, **patch_kw)
# fast algorithm, sigma provided
denoise2_fast = denoise_nl_means(
noisy, h=0.6 * sigma_est, sigma=sigma_est, fast_mode=True, **patch_kw
)
fig, ax = plt.subplots(nrows=2, ncols=3, figsize=(8, 6), sharex=True, sharey=True)
ax[0, 0].imshow(noisy)
ax[0, 0].axis('off')
ax[0, 0].set_title('noisy')
ax[0, 1].imshow(denoise)
ax[0, 1].axis('off')
ax[0, 1].set_title('non-local means\n(slow)')
ax[0, 2].imshow(denoise2)
ax[0, 2].axis('off')
ax[0, 2].set_title('non-local means\n(slow, using $\\sigma_{est}$)')
ax[1, 0].imshow(astro)
ax[1, 0].axis('off')
ax[1, 0].set_title('original\n(noise free)')
ax[1, 1].imshow(denoise_fast)
ax[1, 1].axis('off')
ax[1, 1].set_title('non-local means\n(fast)')
ax[1, 2].imshow(denoise2_fast)
ax[1, 2].axis('off')
ax[1, 2].set_title('non-local means\n(fast, using $\\sigma_{est}$)')
fig.tight_layout()
# print PSNR metric for each case
psnr_noisy = peak_signal_noise_ratio(astro, noisy)
psnr = peak_signal_noise_ratio(astro, denoise)
psnr2 = peak_signal_noise_ratio(astro, denoise2)
psnr_fast = peak_signal_noise_ratio(astro, denoise_fast)
psnr2_fast = peak_signal_noise_ratio(astro, denoise2_fast)
print(f'PSNR (noisy) = {psnr_noisy:0.2f}')
print(f'PSNR (slow) = {psnr:0.2f}')
print(f'PSNR (slow, using sigma) = {psnr2:0.2f}')
print(f'PSNR (fast) = {psnr_fast:0.2f}')
print(f'PSNR (fast, using sigma) = {psnr2_fast:0.2f}')
plt.show()
脚本总运行时间: (0 分钟 0.879 秒)