Note
Go to the end to download the full example code. or to run this example in your browser via Binder
硬币图像的结构化Ward层次聚类演示#
使用Ward层次聚类计算二维图像的分割。聚类在空间上受到约束,以确保每个分割区域都是一个整体。
# 作者:scikit-learn 开发者
# SPDX-License-Identifier:BSD-3-Clause
生成数据#
from skimage.data import coins
orig_coins = coins()
将其调整为原始大小的20%以加快处理速度 在缩小之前应用高斯滤波进行平滑处理 可以减少混叠伪影。
import numpy as np
from scipy.ndimage import gaussian_filter
from skimage.transform import rescale
smoothened_coins = gaussian_filter(orig_coins, sigma=2)
rescaled_coins = rescale(
smoothened_coins,
0.2,
mode="reflect",
anti_aliasing=False,
)
X = np.reshape(rescaled_coins, (-1, 1))
定义数据结构#
像素与其邻居相连。
from sklearn.feature_extraction.image import grid_to_graph
connectivity = grid_to_graph(*rescaled_coins.shape)
计算聚类#
import time as time
from sklearn.cluster import AgglomerativeClustering
print("Compute structured hierarchical clustering...")
st = time.time()
n_clusters = 27 # number of regions
ward = AgglomerativeClustering(
n_clusters=n_clusters, linkage="ward", connectivity=connectivity
)
ward.fit(X)
label = np.reshape(ward.labels_, rescaled_coins.shape)
print(f"Elapsed time: {time.time() - st:.3f}s")
print(f"Number of pixels: {label.size}")
print(f"Number of clusters: {np.unique(label).size}")
Compute structured hierarchical clustering...
Elapsed time: 0.088s
Number of pixels: 4697
Number of clusters: 27
在图像上绘制结果#
凝聚聚类能够分割每个硬币,然而,由于分割在背景中找到了一个较大的区域,我们不得不使用比硬币数量更多的 n_cluster
。
import matplotlib.pyplot as plt
plt.figure(figsize=(5, 5))
plt.imshow(rescaled_coins, cmap=plt.cm.gray)
for l in range(n_clusters):
plt.contour(
label == l,
colors=[
plt.cm.nipy_spectral(l / float(n_clusters)),
],
)
plt.axis("off")
plt.show()
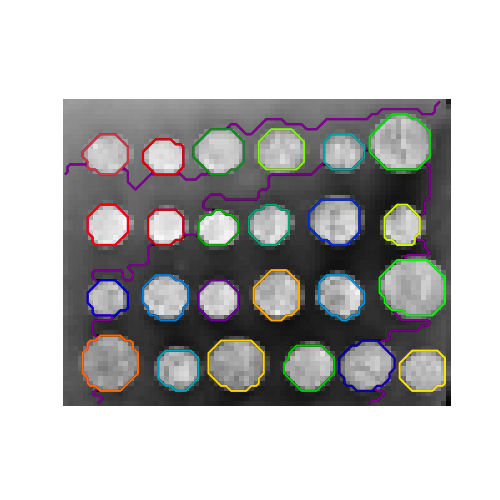
Total running time of the script: (0 minutes 0.146 seconds)
Related examples