Note
Go to the end to download the full example code. or to run this example in your browser via Binder
SVM-Anova:带有单变量特征选择的SVM#
此示例展示了如何在运行SVC(支持向量分类器)之前执行单变量特征选择,以提高分类得分。我们使用鸢尾花数据集(4个特征)并添加36个无信息特征。我们可以发现,当我们选择大约10%的特征时,模型达到了最佳性能。
加载一些数据进行操作#
import numpy as np
from sklearn.datasets import load_iris
X, y = load_iris(return_X_y=True)
# 添加无信息特征
rng = np.random.RandomState(0)
X = np.hstack((X, 2 * rng.random((X.shape[0], 36))))
创建管道#
from sklearn.feature_selection import SelectPercentile, f_classif
from sklearn.pipeline import Pipeline
from sklearn.preprocessing import StandardScaler
from sklearn.svm import SVC
# 创建一个特征选择转换器、一个缩放器和一个SVM实例,将它们组合在一起以构建一个完整的估计器。
clf = Pipeline(
[
("anova", SelectPercentile(f_classif)),
("scaler", StandardScaler()),
("svc", SVC(gamma="auto")),
]
)
绘制交叉验证得分与特征百分位数的关系图#
import matplotlib.pyplot as plt
from sklearn.model_selection import cross_val_score
score_means = list()
score_stds = list()
percentiles = (1, 3, 6, 10, 15, 20, 30, 40, 60, 80, 100)
for percentile in percentiles:
clf.set_params(anova__percentile=percentile)
this_scores = cross_val_score(clf, X, y)
score_means.append(this_scores.mean())
score_stds.append(this_scores.std())
plt.errorbar(percentiles, score_means, np.array(score_stds))
plt.title("Performance of the SVM-Anova varying the percentile of features selected")
plt.xticks(np.linspace(0, 100, 11, endpoint=True))
plt.xlabel("Percentile")
plt.ylabel("Accuracy Score")
plt.axis("tight")
plt.show()
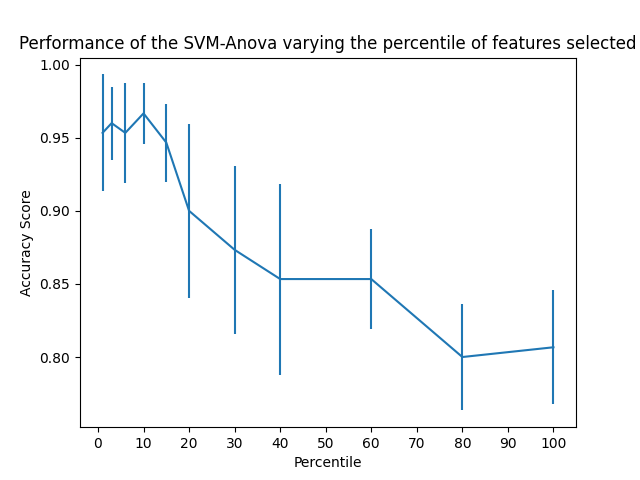
Total running time of the script: (0 minutes 0.136 seconds)
Related examples