Note
Go to the end to download the full example code. or to run this example in your browser via Binder
流形学习方法的比较#
使用各种流形学习方法对S曲线数据集进行降维的示例。
有关这些算法的讨论和比较,请参见 流形模块页面
有关类似的示例,其中这些方法应用于 球面数据集,请参见 球面上的流形学习方法
请注意,MDS的目的是找到数据的低维表示(此处为2D),其中距离很好地反映了原始高维空间中的距离,与其他流形学习算法不同,它不寻求数据在低维空间中的各向同性表示。
# Author: Jake Vanderplas -- <vanderplas@astro.washington.edu>
数据集准备#
我们首先生成S形曲线数据集。
import matplotlib.pyplot as plt
# 未使用但需要导入以使用低于3.2版本的matplotlib进行3D投影
import mpl_toolkits.mplot3d # noqa: F401
from matplotlib import ticker
from sklearn import datasets, manifold
n_samples = 1500
S_points, S_color = datasets.make_s_curve(n_samples, random_state=0)
让我们看看原始数据。同时定义一些辅助函数,我们将在后面使用它们。
def plot_3d(points, points_color, title):
x, y, z = points.T
fig, ax = plt.subplots(
figsize=(6, 6),
facecolor="white",
tight_layout=True,
subplot_kw={"projection": "3d"},
)
fig.suptitle(title, size=16)
col = ax.scatter(x, y, z, c=points_color, s=50, alpha=0.8)
ax.view_init(azim=-60, elev=9)
ax.xaxis.set_major_locator(ticker.MultipleLocator(1))
ax.yaxis.set_major_locator(ticker.MultipleLocator(1))
ax.zaxis.set_major_locator(ticker.MultipleLocator(1))
fig.colorbar(col, ax=ax, orientation="horizontal", shrink=0.6, aspect=60, pad=0.01)
plt.show()
def plot_2d(points, points_color, title):
fig, ax = plt.subplots(figsize=(3, 3), facecolor="white", constrained_layout=True)
fig.suptitle(title, size=16)
add_2d_scatter(ax, points, points_color)
plt.show()
def add_2d_scatter(ax, points, points_color, title=None):
x, y = points.T
ax.scatter(x, y, c=points_color, s=50, alpha=0.8)
ax.set_title(title)
ax.xaxis.set_major_formatter(ticker.NullFormatter())
ax.yaxis.set_major_formatter(ticker.NullFormatter())
plot_3d(S_points, S_color, "Original S-curve samples")
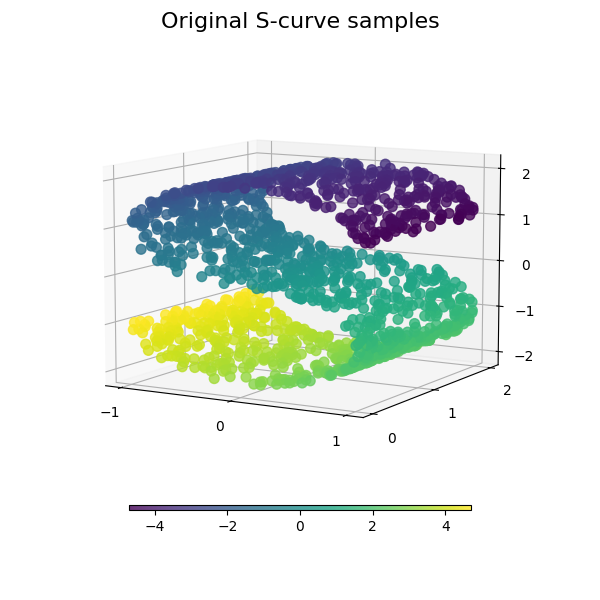
定义流形学习的算法#
流形学习是一种非线性降维方法。用于此任务的算法基于这样一个想法:许多数据集的维度只是人为地高。
请参阅 用户指南 了解更多信息。
n_neighbors = 12 # neighborhood which is used to recover the locally linear structure
n_components = 2 # number of coordinates for the manifold
局部线性嵌入#
局部线性嵌入(LLE)可以看作是一系列局部主成分分析,通过全局比较来找到最佳的非线性嵌入。 在 用户指南 中阅读更多内容。
params = {
"n_neighbors": n_neighbors,
"n_components": n_components,
"eigen_solver": "auto",
"random_state": 0,
}
lle_standard = manifold.LocallyLinearEmbedding(method="standard", **params)
S_standard = lle_standard.fit_transform(S_points)
lle_ltsa = manifold.LocallyLinearEmbedding(method="ltsa", **params)
S_ltsa = lle_ltsa.fit_transform(S_points)
lle_hessian = manifold.LocallyLinearEmbedding(method="hessian", **params)
S_hessian = lle_hessian.fit_transform(S_points)
lle_mod = manifold.LocallyLinearEmbedding(method="modified", **params)
S_mod = lle_mod.fit_transform(S_points)
fig, axs = plt.subplots(
nrows=2, ncols=2, figsize=(7, 7), facecolor="white", constrained_layout=True
)
fig.suptitle("Locally Linear Embeddings", size=16)
lle_methods = [
("Standard locally linear embedding", S_standard),
("Local tangent space alignment", S_ltsa),
("Hessian eigenmap", S_hessian),
("Modified locally linear embedding", S_mod),
]
for ax, method in zip(axs.flat, lle_methods):
name, points = method
add_2d_scatter(ax, points, S_color, name)
plt.show()
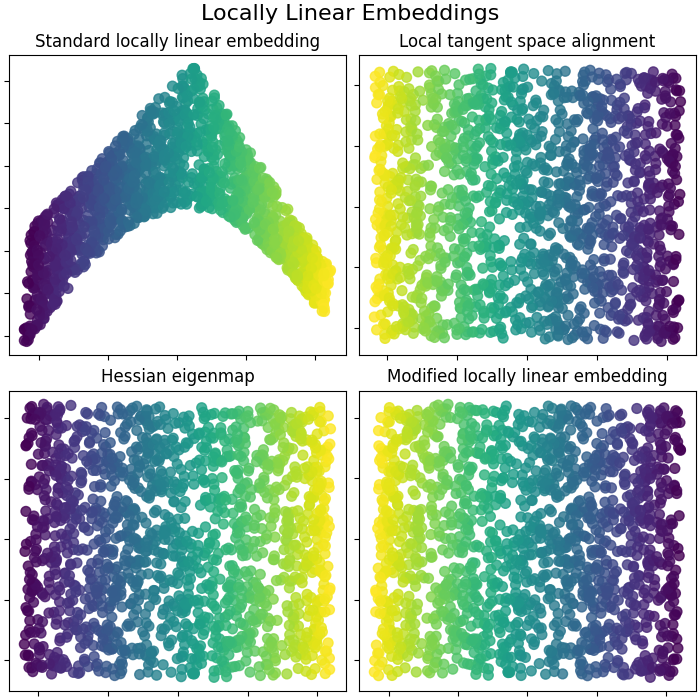
Isomap 嵌入#
通过等距映射进行非线性降维。Isomap 寻求一种低维嵌入,以保持所有点之间的测地距离。更多内容请参阅:用户指南 。
isomap = manifold.Isomap(n_neighbors=n_neighbors, n_components=n_components, p=1)
S_isomap = isomap.fit_transform(S_points)
plot_2d(S_isomap, S_color, "Isomap Embedding")
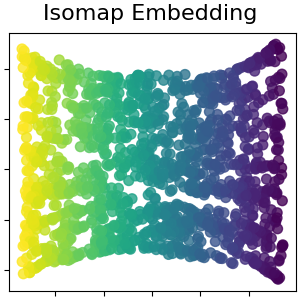
多维尺度分析#
多维尺度分析(MDS)旨在寻找数据的低维表示,其中的距离能够很好地反映原始高维空间中的距离。 更多内容请参阅:用户指南 。
md_scaling = manifold.MDS(
n_components=n_components,
max_iter=50,
n_init=4,
random_state=0,
normalized_stress=False,
)
S_scaling = md_scaling.fit_transform(S_points)
plot_2d(S_scaling, S_color, "Multidimensional scaling")
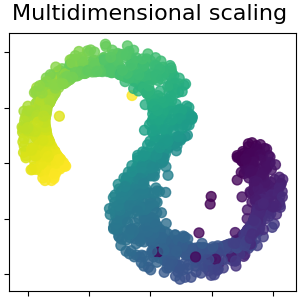
非线性降维的谱嵌入
该实现使用拉普拉斯特征映射,通过图拉普拉斯算子的谱分解找到数据的低维表示。更多内容请参阅:用户指南 。
spectral = manifold.SpectralEmbedding(
n_components=n_components, n_neighbors=n_neighbors, random_state=42
)
S_spectral = spectral.fit_transform(S_points)
plot_2d(S_spectral, S_color, "Spectral Embedding")
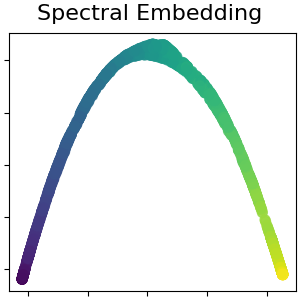
T分布随机邻嵌入
它将数据点之间的相似度转换为联合概率,并尝试最小化低维嵌入和高维数据的联合概率之间的Kullback-Leibler散度。t-SNE的代价函数不是凸的,即不同的初始化可能会得到不同的结果。更多内容请参阅:用户指南 。
t_sne = manifold.TSNE(
n_components=n_components,
perplexity=30,
init="random",
max_iter=250,
random_state=0,
)
S_t_sne = t_sne.fit_transform(S_points)
plot_2d(S_t_sne, S_color, "T-distributed Stochastic \n Neighbor Embedding")
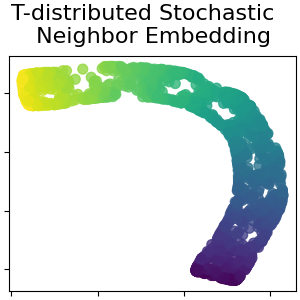
Total running time of the script: (0 minutes 9.337 seconds)
Related examples