Note
Go to the end to download the full example code. or to run this example in your browser via Binder
球面上的流形学习方法#
在球形数据集上应用不同的 流形学习 技术。在这里可以看到使用降维技术来获得关于流形学习方法的一些直觉。关于数据集,球体的两极和侧面的一条薄片被切掉了。这使得流形学习技术能够在将其投影到二维时“展开”它。
对于一个类似的示例,其中这些方法应用于 S 曲线数据集,请参见 流形学习方法的比较
请注意,MDS 的目的是找到数据的低维表示(此处为二维),其中距离很好地尊重原始高维空间中的距离,不同于其他流形学习算法,它不寻求数据在低维空间中的各向同性表示。在这里,流形问题相当于表示地球的平面地图,如 地图投影 。
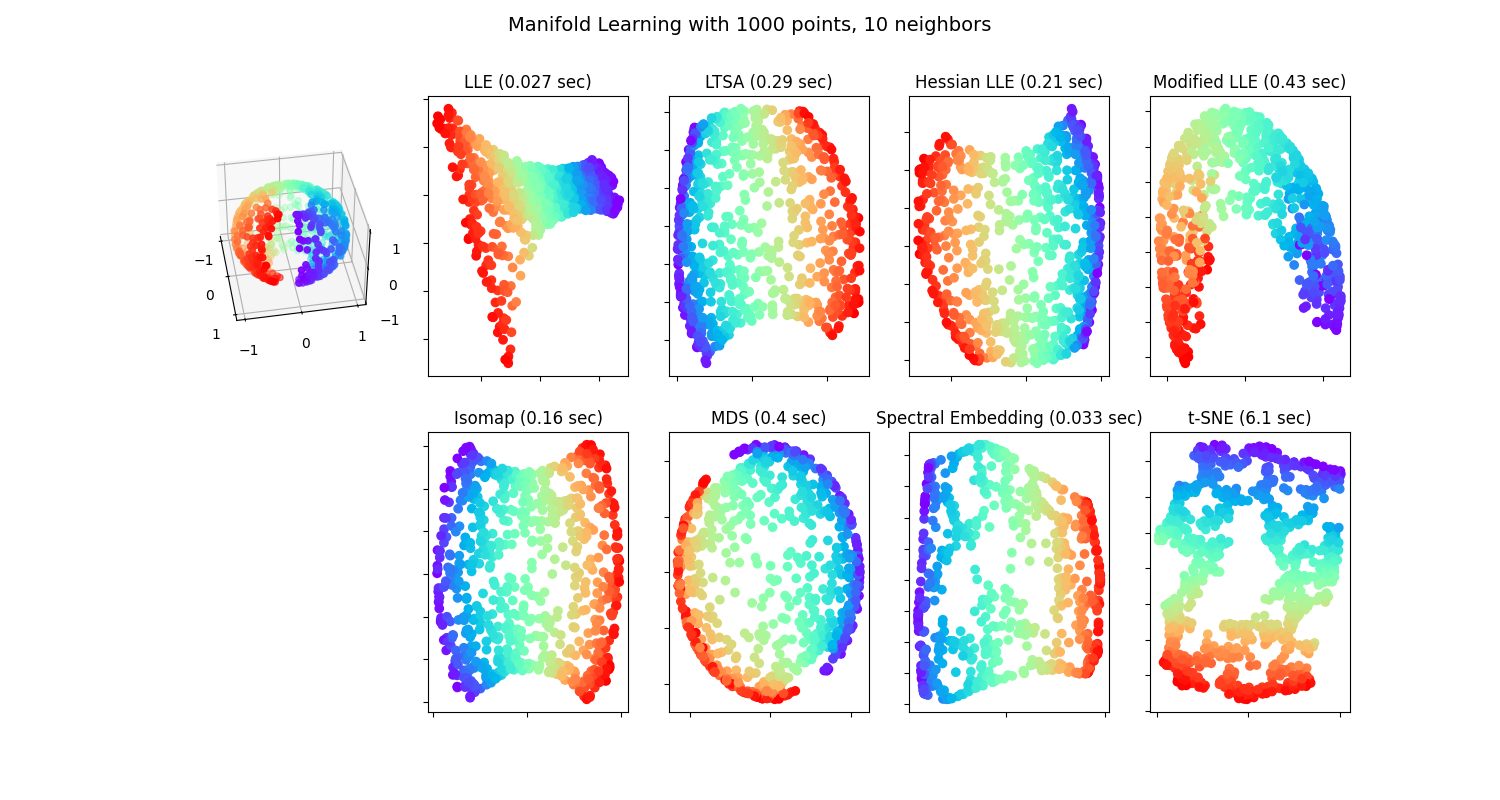
standard: 0.027 sec
ltsa: 0.29 sec
hessian: 0.21 sec
modified: 0.43 sec
ISO: 0.16 sec
MDS: 0.4 sec
Spectral Embedding: 0.033 sec
t-SNE: 6.1 sec
# 作者:scikit-learn 开发者
# SPDX-License-Identifier:BSD-3-Clause
from time import time
import matplotlib.pyplot as plt
# 未使用但需要的导入,用于在 matplotlib < 3.2 中进行 3D 投影
import mpl_toolkits.mplot3d # noqa: F401
import numpy as np
from matplotlib.ticker import NullFormatter
from sklearn import manifold
from sklearn.utils import check_random_state
# 流形学习的变量。
n_neighbors = 10
n_samples = 1000
# 创建我们的球体。
random_state = check_random_state(0)
p = random_state.rand(n_samples) * (2 * np.pi - 0.55)
t = random_state.rand(n_samples) * np.pi
# 将球体的两极切断。
indices = (t < (np.pi - (np.pi / 8))) & (t > ((np.pi / 8)))
colors = p[indices]
x, y, z = (
np.sin(t[indices]) * np.cos(p[indices]),
np.sin(t[indices]) * np.sin(p[indices]),
np.cos(t[indices]),
)
# Plot our dataset.
fig = plt.figure(figsize=(15, 8))
plt.suptitle(
"Manifold Learning with %i points, %i neighbors" % (1000, n_neighbors), fontsize=14
)
ax = fig.add_subplot(251, projection="3d")
ax.scatter(x, y, z, c=p[indices], cmap=plt.cm.rainbow)
ax.view_init(40, -10)
sphere_data = np.array([x, y, z]).T
# 执行局部线性嵌入流形学习
methods = ["standard", "ltsa", "hessian", "modified"]
labels = ["LLE", "LTSA", "Hessian LLE", "Modified LLE"]
for i, method in enumerate(methods):
t0 = time()
trans_data = (
manifold.LocallyLinearEmbedding(
n_neighbors=n_neighbors, n_components=2, method=method, random_state=42
)
.fit_transform(sphere_data)
.T
)
t1 = time()
print("%s: %.2g sec" % (methods[i], t1 - t0))
ax = fig.add_subplot(252 + i)
plt.scatter(trans_data[0], trans_data[1], c=colors, cmap=plt.cm.rainbow)
plt.title("%s (%.2g sec)" % (labels[i], t1 - t0))
ax.xaxis.set_major_formatter(NullFormatter())
ax.yaxis.set_major_formatter(NullFormatter())
plt.axis("tight")
# 执行 Isomap 流形学习。
t0 = time()
trans_data = (
manifold.Isomap(n_neighbors=n_neighbors, n_components=2)
.fit_transform(sphere_data)
.T
)
t1 = time()
print("%s: %.2g sec" % ("ISO", t1 - t0))
ax = fig.add_subplot(257)
plt.scatter(trans_data[0], trans_data[1], c=colors, cmap=plt.cm.rainbow)
plt.title("%s (%.2g sec)" % ("Isomap", t1 - t0))
ax.xaxis.set_major_formatter(NullFormatter())
ax.yaxis.set_major_formatter(NullFormatter())
plt.axis("tight")
# 执行多维缩放。
t0 = time()
mds = manifold.MDS(2, max_iter=100, n_init=1, random_state=42)
trans_data = mds.fit_transform(sphere_data).T
t1 = time()
print("MDS: %.2g sec" % (t1 - t0))
ax = fig.add_subplot(258)
plt.scatter(trans_data[0], trans_data[1], c=colors, cmap=plt.cm.rainbow)
plt.title("MDS (%.2g sec)" % (t1 - t0))
ax.xaxis.set_major_formatter(NullFormatter())
ax.yaxis.set_major_formatter(NullFormatter())
plt.axis("tight")
# 执行谱嵌入。
t0 = time()
se = manifold.SpectralEmbedding(
n_components=2, n_neighbors=n_neighbors, random_state=42
)
trans_data = se.fit_transform(sphere_data).T
t1 = time()
print("Spectral Embedding: %.2g sec" % (t1 - t0))
ax = fig.add_subplot(259)
plt.scatter(trans_data[0], trans_data[1], c=colors, cmap=plt.cm.rainbow)
plt.title("Spectral Embedding (%.2g sec)" % (t1 - t0))
ax.xaxis.set_major_formatter(NullFormatter())
ax.yaxis.set_major_formatter(NullFormatter())
plt.axis("tight")
# 执行t分布随机邻嵌入。
t0 = time()
tsne = manifold.TSNE(n_components=2, random_state=0)
trans_data = tsne.fit_transform(sphere_data).T
t1 = time()
print("t-SNE: %.2g sec" % (t1 - t0))
ax = fig.add_subplot(2, 5, 10)
plt.scatter(trans_data[0], trans_data[1], c=colors, cmap=plt.cm.rainbow)
plt.title("t-SNE (%.2g sec)" % (t1 - t0))
ax.xaxis.set_major_formatter(NullFormatter())
ax.yaxis.set_major_formatter(NullFormatter())
plt.axis("tight")
plt.show()
Total running time of the script: (0 minutes 7.936 seconds)
Related examples