Note
Go to the end to download the full example code. or to run this example in your browser via Binder
交叉验证评分和GridSearchCV的多指标评估演示#
可以通过将 scoring
参数设置为度量标准评分器名称的列表或将评分器名称映射到评分器可调用对象的字典来进行多指标参数搜索。
所有评分器的分数都可以在 cv_results_
字典中以 '_<scorer_name>'
结尾的键中找到(例如 'mean_test_precision'
, 'rank_test_precision'
等)。
best_estimator_
,best_index_
,best_score_
和best_params_
对应于设置为refit
属性的评分器(键)。
# 作者:scikit-learn 开发者
# SPDX-License-Identifier: BSD-3-Clause
import numpy as np
from matplotlib import pyplot as plt
from sklearn.datasets import make_hastie_10_2
from sklearn.metrics import accuracy_score, make_scorer
from sklearn.model_selection import GridSearchCV
from sklearn.tree import DecisionTreeClassifier
使用多种评估指标运行 GridSearchCV
X, y = make_hastie_10_2(n_samples=8000, random_state=42)
# 评分器可以是预定义的度量字符串之一,也可以是一个评分器可调用对象,例如由 make_scorer 返回的对象。
scoring = {"AUC": "roc_auc", "Accuracy": make_scorer(accuracy_score)}
# 将 refit 设置为 'AUC',会使用具有最佳交叉验证 AUC 分数的参数设置在整个数据集上重新拟合估计器。该估计器可以通过 ``gs.best_estimator_`` 获得,同时还包括 ``gs.best_score_`` 、 ``gs.best_params_`` 和 ``gs.best_index_`` 等参数。
gs = GridSearchCV(
DecisionTreeClassifier(random_state=42),
param_grid={"min_samples_split": range(2, 403, 20)},
scoring=scoring,
refit="AUC",
n_jobs=2,
return_train_score=True,
)
gs.fit(X, y)
results = gs.cv_results_
绘制结果#
plt.figure(figsize=(13, 13))
plt.title("GridSearchCV evaluating using multiple scorers simultaneously", fontsize=16)
plt.xlabel("min_samples_split")
plt.ylabel("Score")
ax = plt.gca()
ax.set_xlim(0, 402)
ax.set_ylim(0.73, 1)
# 从MaskedArray中获取常规的numpy数组
X_axis = np.array(results["param_min_samples_split"].data, dtype=float)
for scorer, color in zip(sorted(scoring), ["g", "k"]):
for sample, style in (("train", "--"), ("test", "-")):
sample_score_mean = results["mean_%s_%s" % (sample, scorer)]
sample_score_std = results["std_%s_%s" % (sample, scorer)]
ax.fill_between(
X_axis,
sample_score_mean - sample_score_std,
sample_score_mean + sample_score_std,
alpha=0.1 if sample == "test" else 0,
color=color,
)
ax.plot(
X_axis,
sample_score_mean,
style,
color=color,
alpha=1 if sample == "test" else 0.7,
label="%s (%s)" % (scorer, sample),
)
best_index = np.nonzero(results["rank_test_%s" % scorer] == 1)[0][0]
best_score = results["mean_test_%s" % scorer][best_index]
# 在该评分者的最佳分数处绘制一条由 x 标记的虚线垂直线
ax.plot(
[
X_axis[best_index],
]
* 2,
[0, best_score],
linestyle="-.",
color=color,
marker="x",
markeredgewidth=3,
ms=8,
)
# 为该得分手标注最佳得分
ax.annotate("%0.2f" % best_score, (X_axis[best_index], best_score + 0.005))
plt.legend(loc="best")
plt.grid(False)
plt.show()
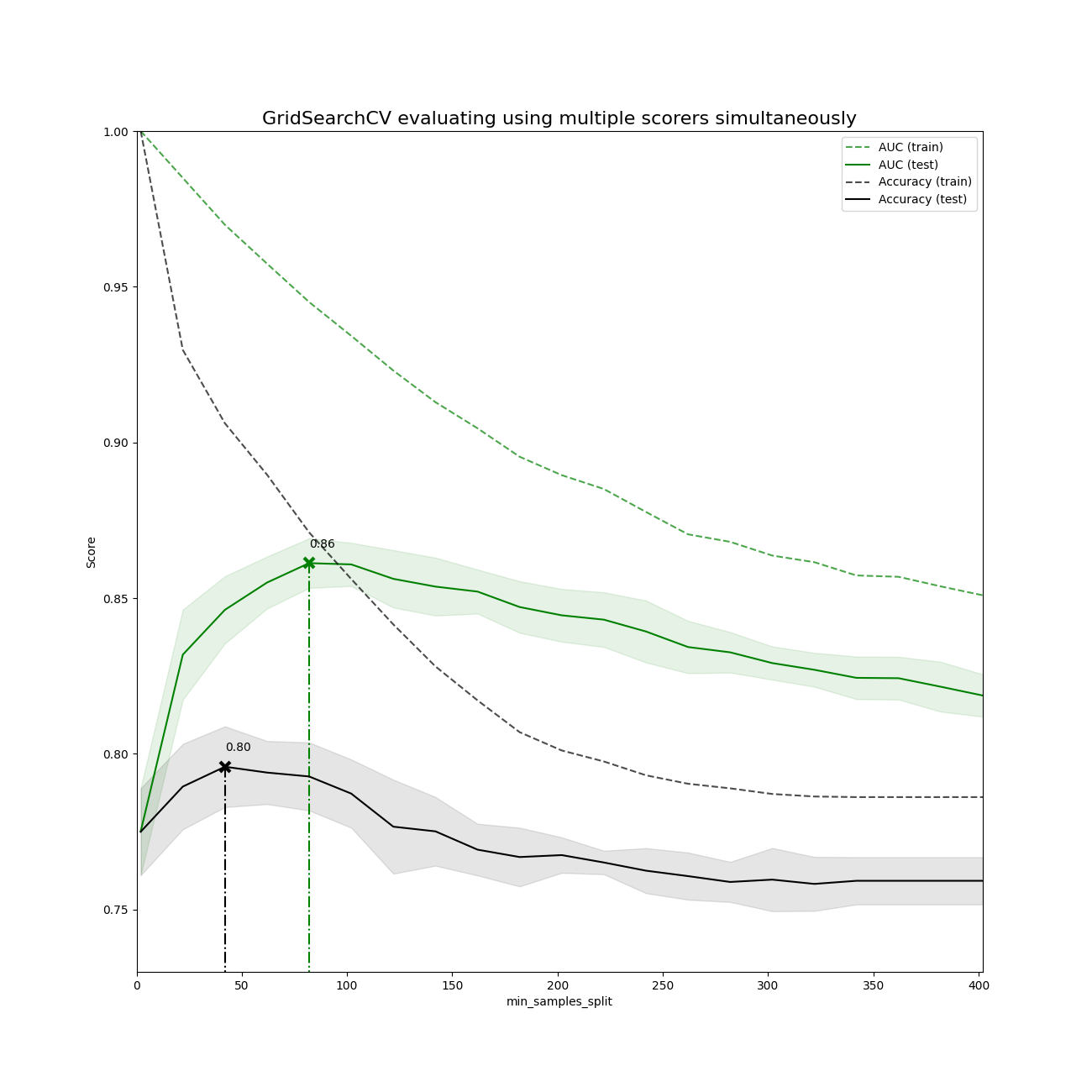
Total running time of the script: (0 minutes 17.010 seconds)
Related examples
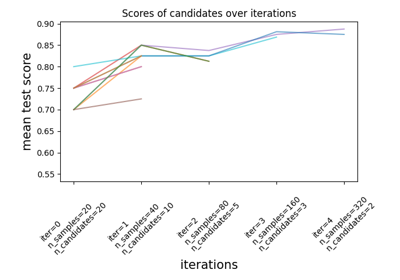
sphx_glr_auto_examples_model_selection_plot_successive_halving_iterations.py
连续减半迭代