Note
Go to the end to download the full example code. or to run this example in your browser via Binder
因子分析(带旋转)以可视化模式#
研究鸢尾花数据集,我们发现花萼长度、花瓣长度和花瓣宽度高度相关。花萼宽度的冗余性较低。矩阵分解技术可以揭示这些潜在模式。对结果组件应用旋转并不会本质上提高衍生潜在空间的预测价值,但可以帮助可视化它们的结构;例如,这里的方差最大化旋转,通过最大化权重的平方方差找到一种结构,其中第二个组件仅在花萼宽度上正加载。
# 作者:scikit-learn 开发者
# SPDX 许可证标识符:BSD-3-Clause
import matplotlib.pyplot as plt
import numpy as np
from sklearn.datasets import load_iris
from sklearn.decomposition import PCA, FactorAnalysis
from sklearn.preprocessing import StandardScaler
%% 加载鸢尾花数据
data = load_iris()
X = StandardScaler().fit_transform(data["data"])
feature_names = data["feature_names"]
绘制鸢尾花特征的协方差
ax = plt.axes()
im = ax.imshow(np.corrcoef(X.T), cmap="RdBu_r", vmin=-1, vmax=1)
ax.set_xticks([0, 1, 2, 3])
ax.set_xticklabels(list(feature_names), rotation=90)
ax.set_yticks([0, 1, 2, 3])
ax.set_yticklabels(list(feature_names))
plt.colorbar(im).ax.set_ylabel("$r$", rotation=0)
ax.set_title("Iris feature correlation matrix")
plt.tight_layout()
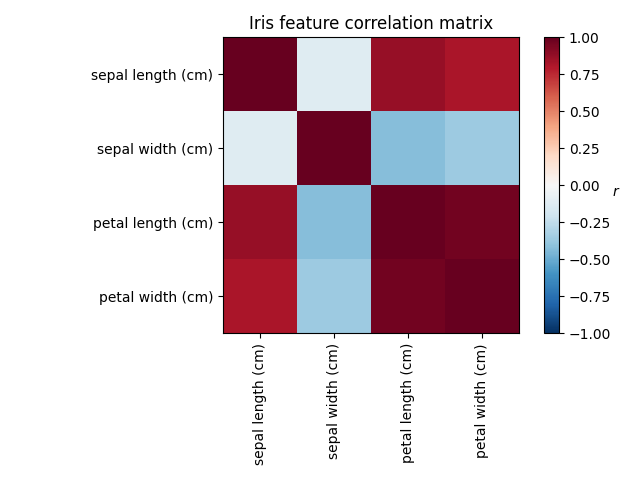
进行带有方差最大化旋转的因子分析
n_comps = 2
methods = [
("PCA", PCA()),
("Unrotated FA", FactorAnalysis()),
("Varimax FA", FactorAnalysis(rotation="varimax")),
]
fig, axes = plt.subplots(ncols=len(methods), figsize=(10, 8), sharey=True)
for ax, (method, fa) in zip(axes, methods):
fa.set_params(n_components=n_comps)
fa.fit(X)
components = fa.components_.T
print("\n\n %s :\n" % method)
print(components)
vmax = np.abs(components).max()
ax.imshow(components, cmap="RdBu_r", vmax=vmax, vmin=-vmax)
ax.set_yticks(np.arange(len(feature_names)))
ax.set_yticklabels(feature_names)
ax.set_title(str(method))
ax.set_xticks([0, 1])
ax.set_xticklabels(["Comp. 1", "Comp. 2"])
fig.suptitle("Factors")
plt.tight_layout()
plt.show()
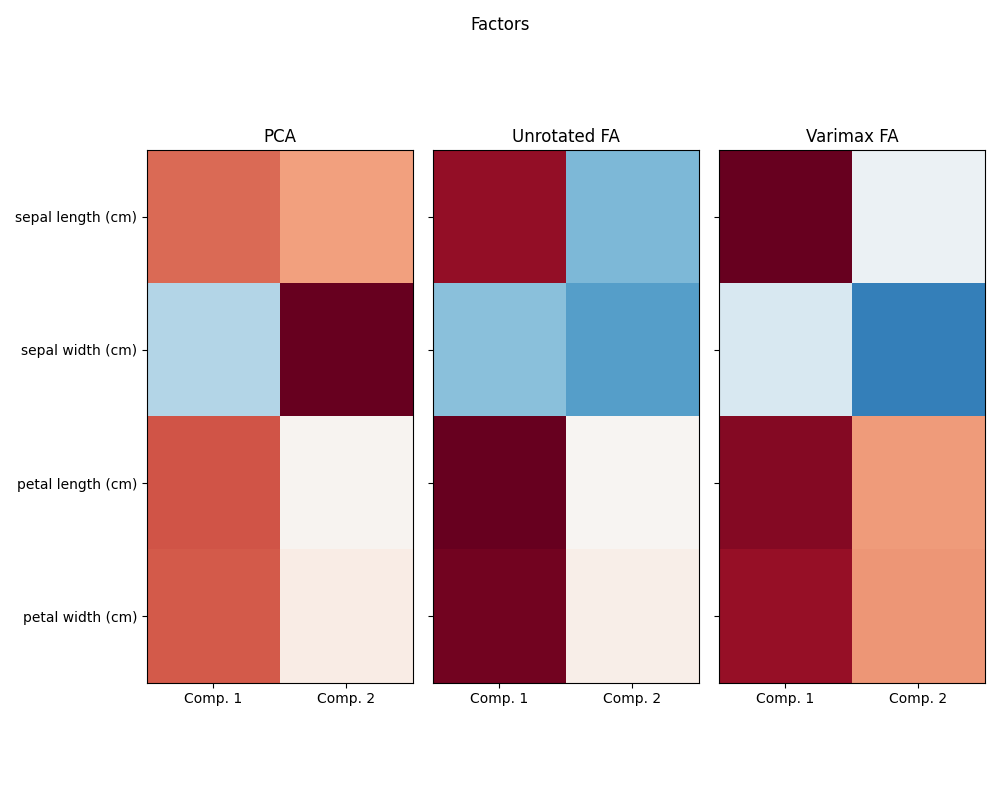
PCA :
[[ 0.52106591 0.37741762]
[-0.26934744 0.92329566]
[ 0.5804131 0.02449161]
[ 0.56485654 0.06694199]]
Unrotated FA :
[[ 0.88096009 -0.4472869 ]
[-0.41691605 -0.55390036]
[ 0.99918858 0.01915283]
[ 0.96228895 0.05840206]]
Varimax FA :
[[ 0.98633022 -0.05752333]
[-0.16052385 -0.67443065]
[ 0.90809432 0.41726413]
[ 0.85857475 0.43847489]]
Total running time of the script: (0 minutes 0.239 seconds)
Related examples