Note
Go to the end to download the full example code. or to run this example in your browser via Binder
线性模型系数解释中的常见陷阱#
在线性模型中,目标值被建模为特征的线性组合(有关scikit-learn中可用的一组线性模型的描述,请参见:ref:linear_model
用户指南部分)。多重线性模型中的系数表示给定特征:math:X_i
和目标:math:y
之间的关系,假设所有其他特征保持不变( 条件依赖性 )。这与绘制:math:X_i
对:math:y
并拟合线性关系不同:在那种情况下,估计中考虑了所有其他特征的可能值(边际依赖性)。
本示例将提供一些解释线性模型中系数的提示,指出当线性模型不适合描述数据集或特征相关时出现的问题。
Note
请记住,特征:math:X
和结果:math:y
通常是我们未知的数据生成过程的结果。机器学习模型经过训练以从样本数据中逼近将:math:X
与:math:y
链接的未观察到的数学函数。因此,对模型所做的任何解释可能不一定能推广到真实的数据生成过程。当模型质量较差或样本数据不代表总体时,这一点尤其正确。
我们将使用1985年的 “当前人口调查” 数据来预测工资,作为经验、年龄或教育等各种特征的函数。
import matplotlib.pyplot as plt
import numpy as np
import pandas as pd
import scipy as sp
import seaborn as sns
数据集:工资#
我们从 OpenML 获取数据。
请注意,将参数 as_frame
设置为 True 会将数据检索为 pandas dataframe。
from sklearn.datasets import fetch_openml
survey = fetch_openml(data_id=534, as_frame=True)
然后,我们确定特征 X
和目标 y
:列 WAGE 是我们的目标变量(即我们想要预测的变量)。
X = survey.data[survey.feature_names]
X.describe(include="all")
请注意,数据集包含分类变量和数值变量。在之后的预处理中,我们需要考虑这一点。
X.head()
我们的预测目标:工资。 工资以每小时美元为单位,描述为浮点数。
y = survey.target.values.ravel()
survey.target.head()
0 5.10
1 4.95
2 6.67
3 4.00
4 7.50
Name: WAGE, dtype: float64
我们将样本分为训练集和测试集。 在接下来的探索性分析中只会使用训练集。 这是模拟在真实情况下对未知目标进行预测的一种方法,我们不希望我们的分析和决策因了解测试数据而产生偏差。
from sklearn.model_selection import train_test_split
X_train, X_test, y_train, y_test = train_test_split(X, y, random_state=42)
首先,让我们通过查看变量分布和它们之间的成对关系来获取一些见解。只使用数值变量。在下图中,每个点代表一个样本。
train_dataset = X_train.copy()
train_dataset.insert(0, "WAGE", y_train)
_ = sns.pairplot(train_dataset, kind="reg", diag_kind="kde")
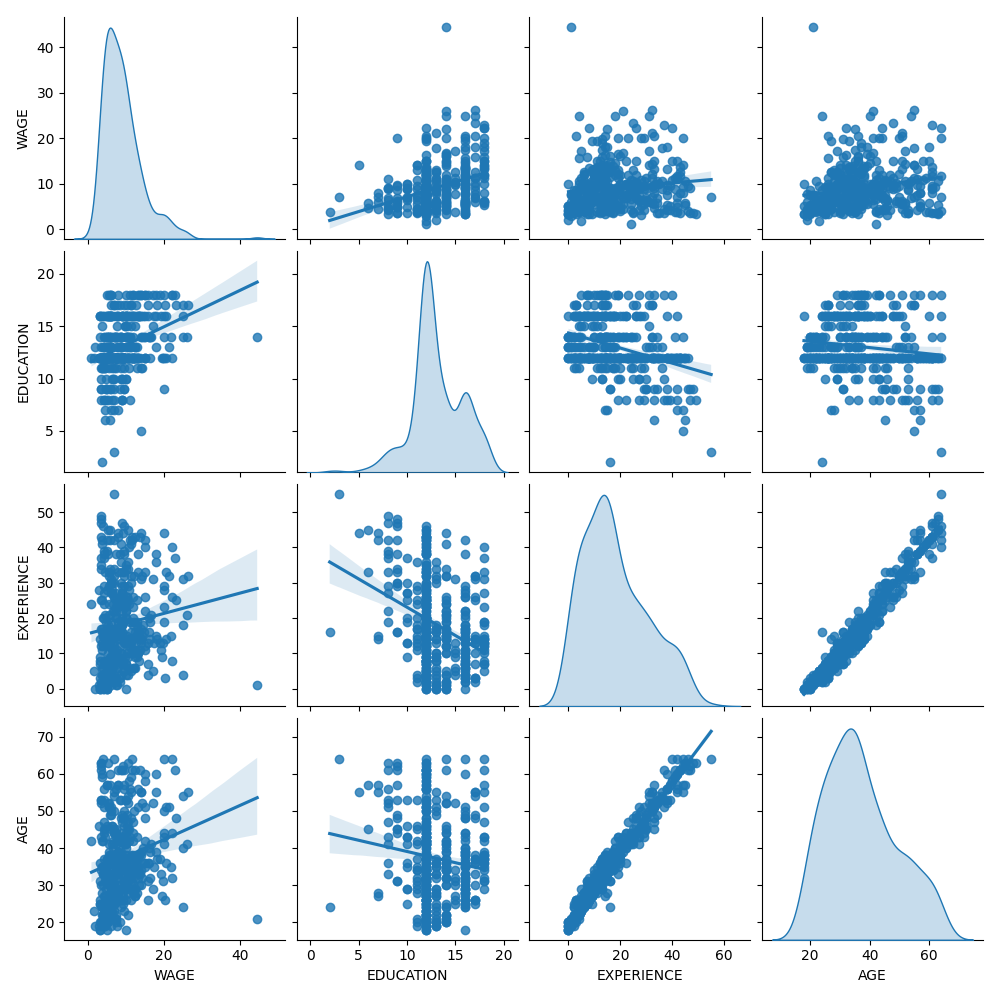
仔细观察工资分布会发现它有一个长尾。因此,我们应该取其对数,将其大致转化为正态分布(线性模型如岭回归或套索回归在误差呈正态分布时效果最佳)。
工资随着教育水平的提高而增加。请注意,这里表示的工资和教育之间的依赖关系是边际依赖关系,即描述特定变量的行为而不固定其他变量。
此外,经验和年龄之间存在强烈的线性相关性。
机器学习流水线#
为了设计我们的机器学习管道,我们首先手动检查我们正在处理的数据类型:
survey.data.info()
<class 'pandas.core.frame.DataFrame'>
RangeIndex: 534 entries, 0 to 533
Data columns (total 10 columns):
# Column Non-Null Count Dtype
--- ------ -------------- -----
0 EDUCATION 534 non-null int64
1 SOUTH 534 non-null category
2 SEX 534 non-null category
3 EXPERIENCE 534 non-null int64
4 UNION 534 non-null category
5 AGE 534 non-null int64
6 RACE 534 non-null category
7 OCCUPATION 534 non-null category
8 SECTOR 534 non-null category
9 MARR 534 non-null category
dtypes: category(7), int64(3)
memory usage: 17.3 KB
正如之前所见,数据集包含具有不同数据类型的列,我们需要对每种数据类型应用特定的预处理。特别是,如果分类变量不先编码为整数,则不能将其包含在线性模型中。此外,为了避免将分类特征视为有序值,我们需要对其进行独热编码。我们的预处理器将会
对类别列进行独热编码(即按类别生成列),仅对非二元类别变量进行;
作为第一种方法(之后我们将讨论数值归一化如何影响我们的讨论),保持数值不变。
from sklearn.compose import make_column_transformer
from sklearn.preprocessing import OneHotEncoder
categorical_columns = ["RACE", "OCCUPATION", "SECTOR", "MARR", "UNION", "SEX", "SOUTH"]
numerical_columns = ["EDUCATION", "EXPERIENCE", "AGE"]
preprocessor = make_column_transformer(
(OneHotEncoder(drop="if_binary"), categorical_columns),
remainder="passthrough",
verbose_feature_names_out=False, # avoid to prepend the preprocessor names
)
为了将数据集描述为线性模型,我们使用带有非常小正则化的岭回归器,并对工资的对数进行建模。
from sklearn.compose import TransformedTargetRegressor
from sklearn.linear_model import Ridge
from sklearn.pipeline import make_pipeline
model = make_pipeline(
preprocessor,
TransformedTargetRegressor(
regressor=Ridge(alpha=1e-10), func=np.log10, inverse_func=sp.special.exp10
),
)
处理数据集#
首先,我们拟合模型。
model.fit(X_train, y_train)
然后我们通过在测试集上绘制预测结果并计算模型的性能,例如计算模型的中位绝对误差,来检查计算模型的性能。
from sklearn.metrics import PredictionErrorDisplay, median_absolute_error
mae_train = median_absolute_error(y_train, model.predict(X_train))
y_pred = model.predict(X_test)
mae_test = median_absolute_error(y_test, y_pred)
scores = {
"MedAE on training set": f"{mae_train:.2f} $/hour",
"MedAE on testing set": f"{mae_test:.2f} $/hour",
}
_, ax = plt.subplots(figsize=(5, 5))
display = PredictionErrorDisplay.from_predictions(
y_test, y_pred, kind="actual_vs_predicted", ax=ax, scatter_kwargs={"alpha": 0.5}
)
ax.set_title("Ridge model, small regularization")
for name, score in scores.items():
ax.plot([], [], " ", label=f"{name}: {score}")
ax.legend(loc="upper left")
plt.tight_layout()
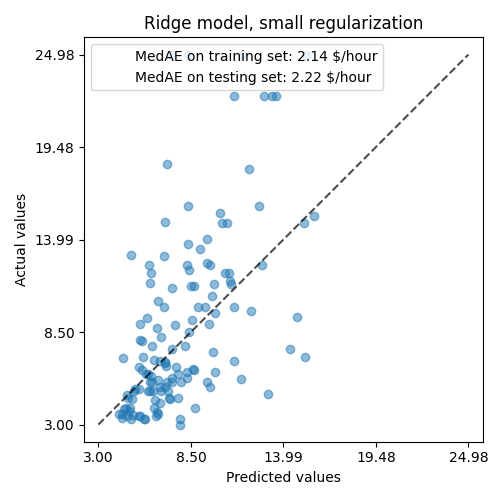
模型学习结果远未成为一个能够做出准确预测的好模型: 这一点在上面的图表中显而易见,好的预测应该位于黑色虚线附近。
在接下来的部分中,我们将解释模型的系数。在此过程中,我们应牢记,任何结论都是关于我们构建的模型,而非关于数据的真实(现实世界)生成过程。
解释系数:尺度很重要#
首先,我们可以查看我们拟合的回归模型的系数值。
feature_names = model[:-1].get_feature_names_out()
coefs = pd.DataFrame(
model[-1].regressor_.coef_,
columns=["Coefficients"],
index=feature_names,
)
coefs
AGE 系数以“美元/小时每生存年”表示,而 EDUCATION 系数以“美元/小时每教育年”表示。这种系数表示方式的好处在于可以清楚地展示模型的实际预测:AGE 增加 1 年意味着每小时减少 0.030867 美元,而 EDUCATION 增加 1 年意味着每小时增加 0.054699 美元。另一方面,分类变量(如 UNION 或 SEX)是无量纲的数字,取值为 0 或 1。它们的系数以美元/小时表示。因此,我们不能比较不同系数的大小,因为特征具有不同的自然尺度,因此由于度量单位不同,其取值范围也不同。如果我们绘制系数,这一点会更加明显。
coefs.plot.barh(figsize=(9, 7))
plt.title("Ridge model, small regularization")
plt.axvline(x=0, color=".5")
plt.xlabel("Raw coefficient values")
plt.subplots_adjust(left=0.3)
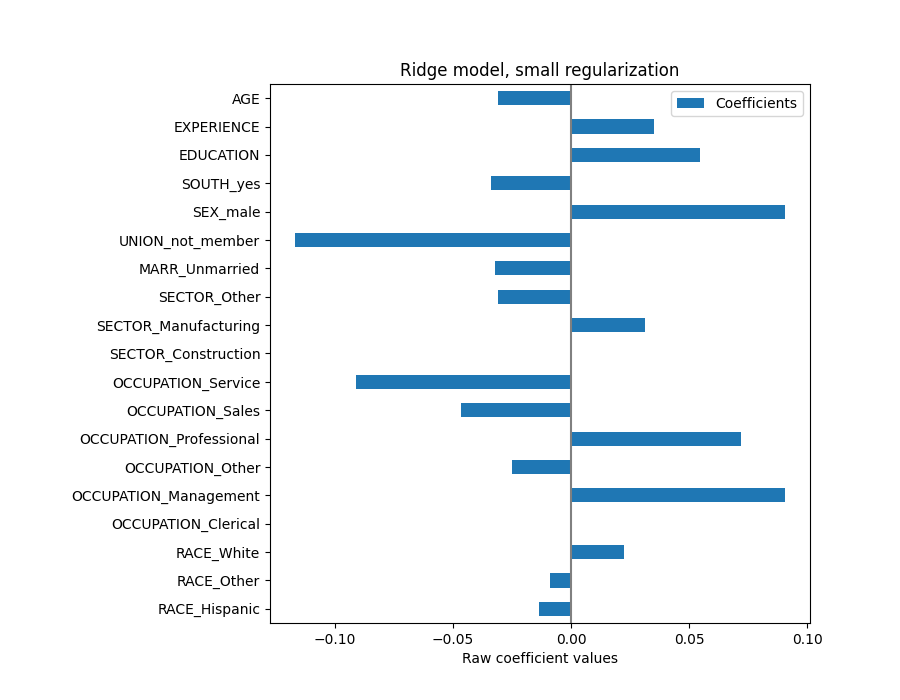
事实上,从上面的图表来看,决定工资的最重要因素似乎是变量UNION,即使我们的直觉可能会告诉我们,像EXPERIENCE这样的变量应该有更大的影响。
查看系数图以评估特征重要性可能会产生误导,因为其中一些特征的变化范围很小,而另一些特征(如年龄)的变化范围要大得多,可能跨越数十年。
如果我们比较不同特征的标准差,这一点就很明显。
X_train_preprocessed = pd.DataFrame(
model[:-1].transform(X_train), columns=feature_names
)
X_train_preprocessed.std(axis=0).plot.barh(figsize=(9, 7))
plt.title("Feature ranges")
plt.xlabel("Std. dev. of feature values")
plt.subplots_adjust(left=0.3)
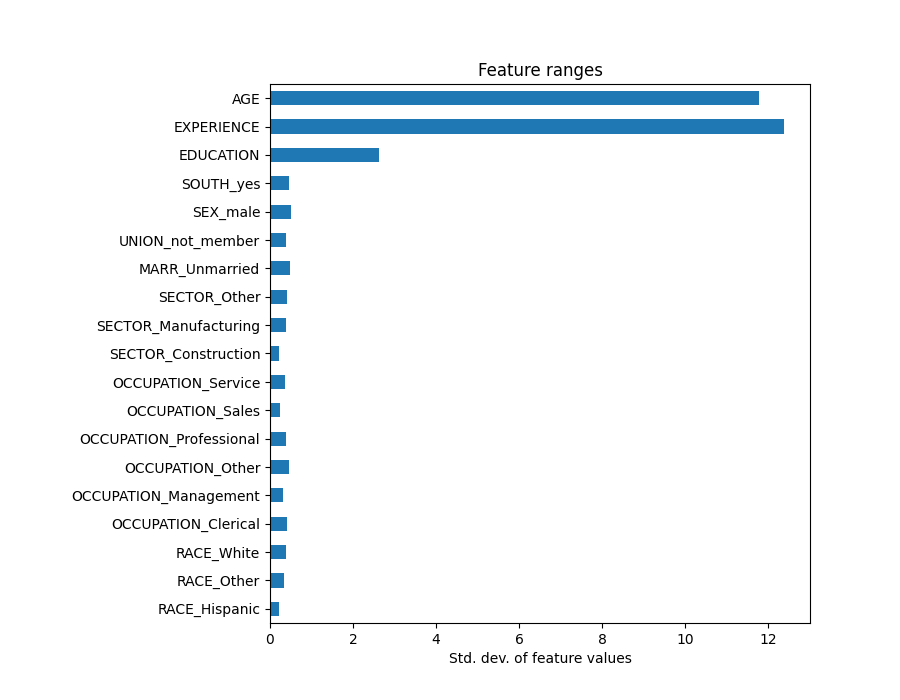
将系数乘以相关特征的标准差会将所有系数减少到相同的度量单位。正如我们将在 后面 看到的,这相当于将数值变量标准化为它们的标准差,如
通过这种方式,我们强调了一个特征的方差越大,在其他条件相同的情况下,相应系数对输出的权重就越大。
coefs = pd.DataFrame(
model[-1].regressor_.coef_ * X_train_preprocessed.std(axis=0),
columns=["Coefficient importance"],
index=feature_names,
)
coefs.plot(kind="barh", figsize=(9, 7))
plt.xlabel("Coefficient values corrected by the feature's std. dev.")
plt.title("Ridge model, small regularization")
plt.axvline(x=0, color=".5")
plt.subplots_adjust(left=0.3)
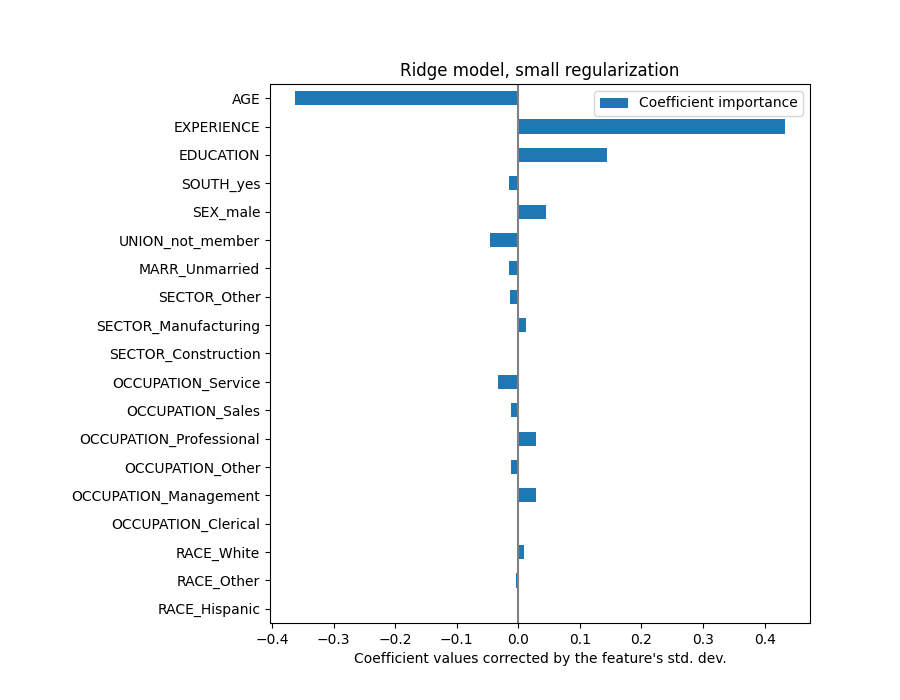
现在系数已经被缩放,我们可以安全地比较它们了。
为什么上面的图表表明年龄增加会导致工资下降?为什么 initial pairplot 显示相反的结果?
上图告诉我们,当所有其他特征保持不变时,特定特征与目标之间的依赖关系,即 条件依赖 。当所有其他特征保持不变时,AGE 的增加会导致 WAGE 的减少。相反,当所有其他特征保持不变时,EXPERIENCE 的增加会导致 WAGE 的增加。此外,AGE、EXPERIENCE 和 EDUCATION 是对模型影响最大的三个变量。
解释系数:谨慎对待因果关系#
线性模型是衡量统计关联的一个很好的工具,但在做因果关系陈述时我们应该谨慎,毕竟相关性并不总是意味着因果关系。这在社会科学中尤其困难,因为我们观察到的变量仅作为潜在因果过程的代理。
在我们的特定情况下,我们可以将个人的教育视为其专业能力的替代指标,这是我们感兴趣但无法直接观察的真实变量。我们当然希望认为在校时间越长会提高技术能力,但也很有可能因果关系是相反的。也就是说,技术能力强的人往往会在校时间更长。
一个雇主不太可能在意是哪种情况(或者两者混合),只要他们仍然相信受教育程度更高的人更适合这份工作,他们就会乐意支付更高的工资。
当考虑某种形式的干预措施时,例如政府对大学学位的补贴或鼓励个人接受高等教育的宣传材料,这种效应的混淆会变得有问题。特别是如果混淆程度很高,这些措施的有效性可能会被夸大。我们的模型预测每增加一年教育,时薪会增加
检查系数的变异性#
我们可以通过交叉验证检查系数的变异性: 这是一种数据扰动形式(与 重采样 相关)。
如果在更换输入数据集时系数变化显著,则其稳健性无法得到保证,可能需要谨慎解释。
from sklearn.model_selection import RepeatedKFold, cross_validate
cv = RepeatedKFold(n_splits=5, n_repeats=5, random_state=0)
cv_model = cross_validate(
model,
X,
y,
cv=cv,
return_estimator=True,
n_jobs=2,
)
coefs = pd.DataFrame(
[
est[-1].regressor_.coef_ * est[:-1].transform(X.iloc[train_idx]).std(axis=0)
for est, (train_idx, _) in zip(cv_model["estimator"], cv.split(X, y))
],
columns=feature_names,
)
plt.figure(figsize=(9, 7))
sns.stripplot(data=coefs, orient="h", palette="dark:k", alpha=0.5)
sns.boxplot(data=coefs, orient="h", color="cyan", saturation=0.5, whis=10)
plt.axvline(x=0, color=".5")
plt.xlabel("Coefficient importance")
plt.title("Coefficient importance and its variability")
plt.suptitle("Ridge model, small regularization")
plt.subplots_adjust(left=0.3)
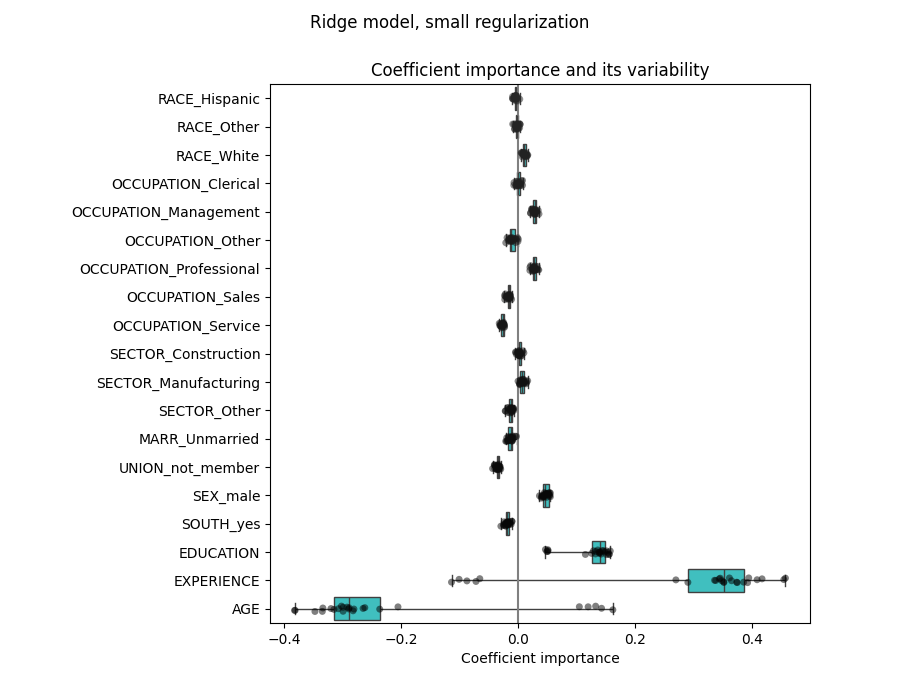
变量相关性问题#
AGE 和 EXPERIENCE 系数受强烈变异的影响,这可能是由于这两个特征之间的共线性:由于 AGE 和 EXPERIENCE 在数据中一起变化,它们的影响难以区分。
为了验证这一解释,我们绘制了AGE和EXPERIENCE系数的变异性。
plt.ylabel("Age coefficient")
plt.xlabel("Experience coefficient")
plt.grid(True)
plt.xlim(-0.4, 0.5)
plt.ylim(-0.4, 0.5)
plt.scatter(coefs["AGE"], coefs["EXPERIENCE"])
_ = plt.title("Co-variations of coefficients for AGE and EXPERIENCE across folds")
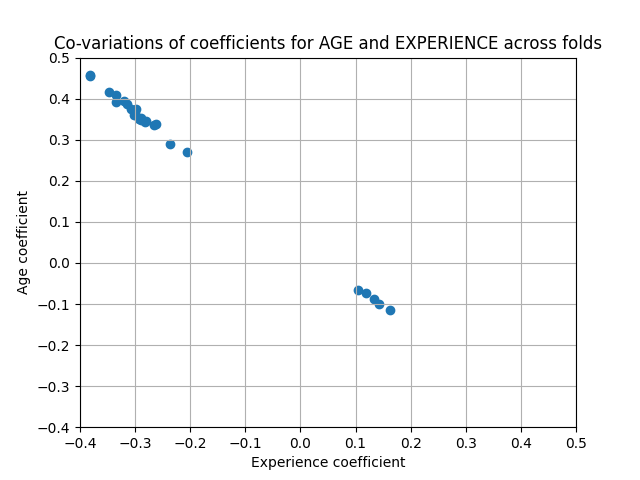
两个区域被填充:当EXPERIENCE系数为正时,AGE系数为负,反之亦然。
为了进一步研究,我们移除两个特征中的一个,并检查其对模型稳定性的影响。
column_to_drop = ["AGE"]
cv_model = cross_validate(
model,
X.drop(columns=column_to_drop),
y,
cv=cv,
return_estimator=True,
n_jobs=2,
)
coefs = pd.DataFrame(
[
est[-1].regressor_.coef_
* est[:-1].transform(X.drop(columns=column_to_drop).iloc[train_idx]).std(axis=0)
for est, (train_idx, _) in zip(cv_model["estimator"], cv.split(X, y))
],
columns=feature_names[:-1],
)
plt.figure(figsize=(9, 7))
sns.stripplot(data=coefs, orient="h", palette="dark:k", alpha=0.5)
sns.boxplot(data=coefs, orient="h", color="cyan", saturation=0.5)
plt.axvline(x=0, color=".5")
plt.title("Coefficient importance and its variability")
plt.xlabel("Coefficient importance")
plt.suptitle("Ridge model, small regularization, AGE dropped")
plt.subplots_adjust(left=0.3)
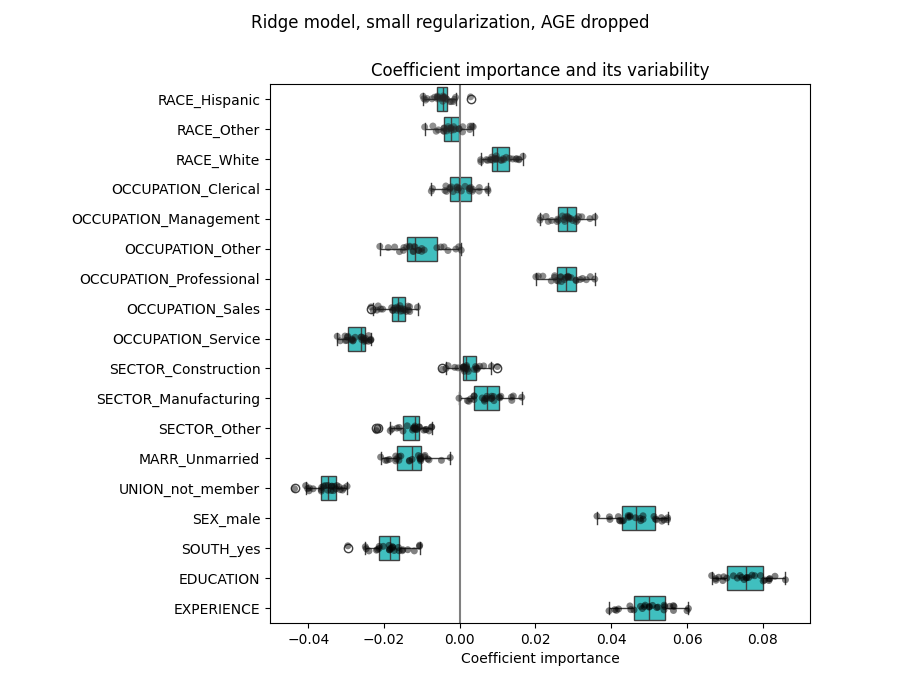
EXPERIENCE 系数的估计现在显示出更小的变异性。EXPERIENCE 在交叉验证期间训练的所有模型中仍然很重要。
对数值变量进行预处理#
如上所述(参见 “机器学习流水线 “),我们也可以选择在训练模型之前对数值进行缩放。 当我们对所有数值应用相似量的正则化时,这可能会很有用。 重新定义预处理器以减去均值并将变量缩放到单位方差。
from sklearn.preprocessing import StandardScaler
preprocessor = make_column_transformer(
(OneHotEncoder(drop="if_binary"), categorical_columns),
(StandardScaler(), numerical_columns),
)
模型将保持不变。
model = make_pipeline(
preprocessor,
TransformedTargetRegressor(
regressor=Ridge(alpha=1e-10), func=np.log10, inverse_func=sp.special.exp10
),
)
model.fit(X_train, y_train)
我们再次检查计算模型的性能,例如使用模型的中位绝对误差和R平方系数。
mae_train = median_absolute_error(y_train, model.predict(X_train))
y_pred = model.predict(X_test)
mae_test = median_absolute_error(y_test, y_pred)
scores = {
"MedAE on training set": f"{mae_train:.2f} $/hour",
"MedAE on testing set": f"{mae_test:.2f} $/hour",
}
_, ax = plt.subplots(figsize=(5, 5))
display = PredictionErrorDisplay.from_predictions(
y_test, y_pred, kind="actual_vs_predicted", ax=ax, scatter_kwargs={"alpha": 0.5}
)
ax.set_title("Ridge model, small regularization")
for name, score in scores.items():
ax.plot([], [], " ", label=f"{name}: {score}")
ax.legend(loc="upper left")
plt.tight_layout()
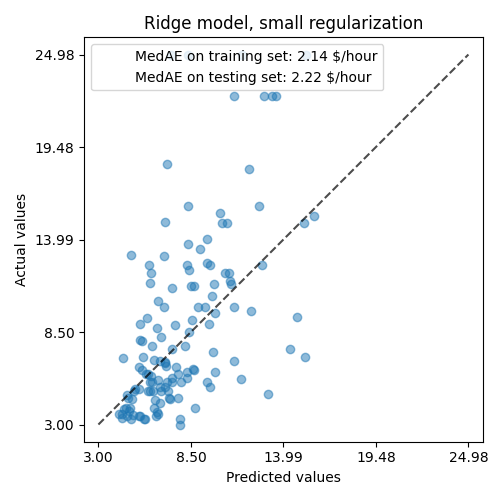
对于系数分析,这次不需要进行缩放,因为在预处理步骤中已经完成了。
coefs = pd.DataFrame(
model[-1].regressor_.coef_,
columns=["Coefficients importance"],
index=feature_names,
)
coefs.plot.barh(figsize=(9, 7))
plt.title("Ridge model, small regularization, normalized variables")
plt.xlabel("Raw coefficient values")
plt.axvline(x=0, color=".5")
plt.subplots_adjust(left=0.3)
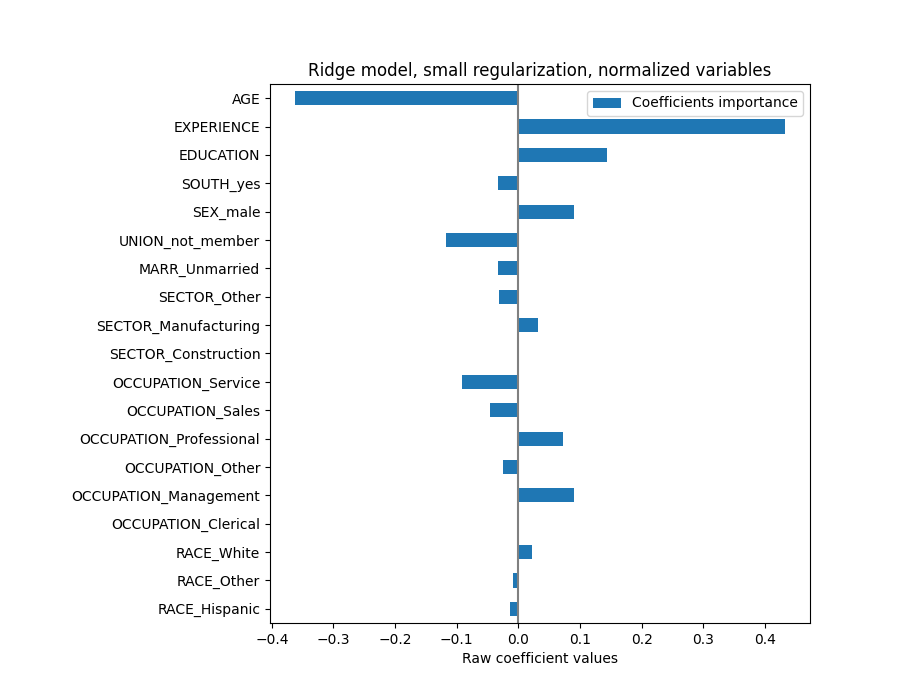
我们现在检查几个交叉验证折叠中的系数。与上面的例子一样,我们不需要通过特征值的标准差来缩放系数,因为这种缩放已经在管道的预处理步骤中完成了。
cv_model = cross_validate(
model,
X,
y,
cv=cv,
return_estimator=True,
n_jobs=2,
)
coefs = pd.DataFrame(
[est[-1].regressor_.coef_ for est in cv_model["estimator"]], columns=feature_names
)
plt.figure(figsize=(9, 7))
sns.stripplot(data=coefs, orient="h", palette="dark:k", alpha=0.5)
sns.boxplot(data=coefs, orient="h", color="cyan", saturation=0.5, whis=10)
plt.axvline(x=0, color=".5")
plt.title("Coefficient variability")
plt.subplots_adjust(left=0.3)
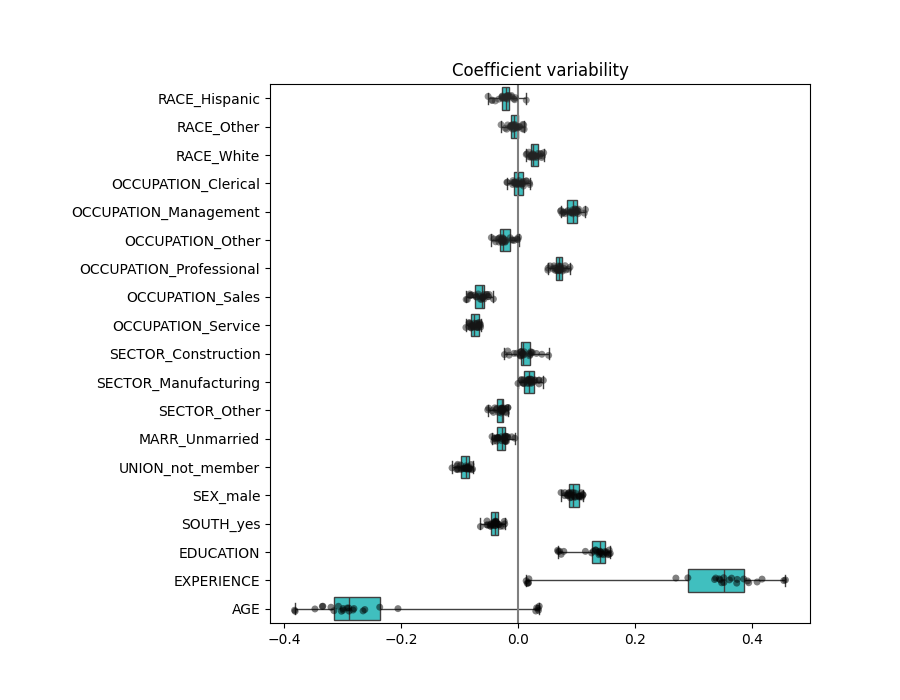
结果与非标准化情况非常相似。
线性模型与正则化#
在机器学习实践中,岭回归更常用于非可忽略的正则化。
在上文中,我们将正则化限制在一个非常小的量。正则化改善了问题的条件并减少了估计的方差。RidgeCV
应用交叉验证来确定哪个正则化参数( alpha
)的值最适合用于预测。
from sklearn.linear_model import RidgeCV
alphas = np.logspace(-10, 10, 21) # alpha values to be chosen from by cross-validation
model = make_pipeline(
preprocessor,
TransformedTargetRegressor(
regressor=RidgeCV(alphas=alphas),
func=np.log10,
inverse_func=sp.special.exp10,
),
)
model.fit(X_train, y_train)
首先,我们检查选择了哪个
model[-1].regressor_.alpha_
np.float64(10.0)
然后我们检查预测的质量。
mae_train = median_absolute_error(y_train, model.predict(X_train))
y_pred = model.predict(X_test)
mae_test = median_absolute_error(y_test, y_pred)
scores = {
"MedAE on training set": f"{mae_train:.2f} $/hour",
"MedAE on testing set": f"{mae_test:.2f} $/hour",
}
_, ax = plt.subplots(figsize=(5, 5))
display = PredictionErrorDisplay.from_predictions(
y_test, y_pred, kind="actual_vs_predicted", ax=ax, scatter_kwargs={"alpha": 0.5}
)
ax.set_title("Ridge model, optimum regularization")
for name, score in scores.items():
ax.plot([], [], " ", label=f"{name}: {score}")
ax.legend(loc="upper left")
plt.tight_layout()
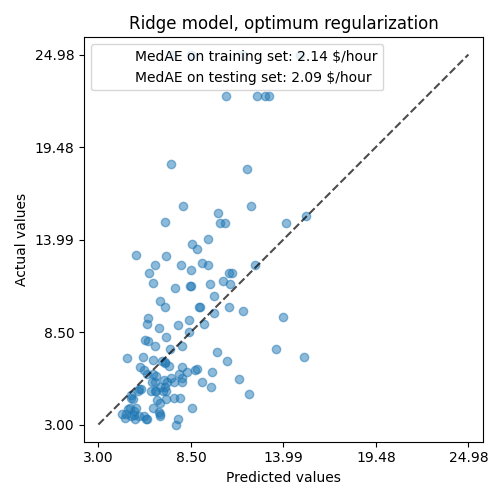
正则化模型重现数据的能力与非正则化模型相似。
coefs = pd.DataFrame(
model[-1].regressor_.coef_,
columns=["Coefficients importance"],
index=feature_names,
)
coefs.plot.barh(figsize=(9, 7))
plt.title("Ridge model, with regularization, normalized variables")
plt.xlabel("Raw coefficient values")
plt.axvline(x=0, color=".5")
plt.subplots_adjust(left=0.3)
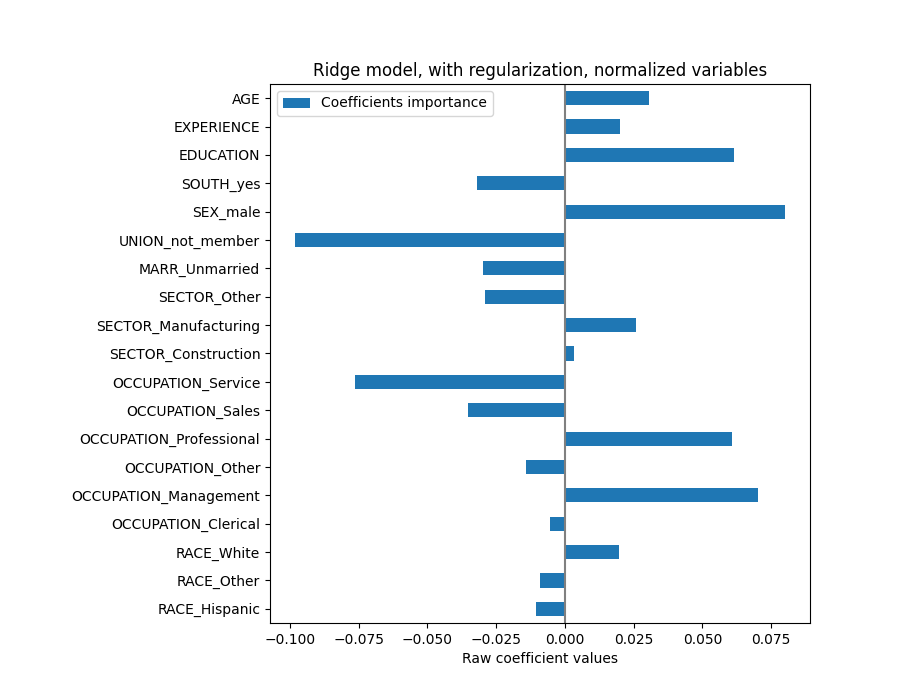
系数显著不同。 AGE 和 EXPERIENCE 系数都是正的,但它们对预测的影响现在较小。
正则化减少了相关变量对模型的影响,因为权重在两个预测变量之间共享,因此单独一个变量的权重都不会很大。
另一方面,通过正则化获得的权重更加稳定(参见 岭回归和分类 用户指南部分)。这种稳定性的提高可以从交叉验证中数据扰动得到的图中看出。该图可以与 前一个 进行比较。
cv_model = cross_validate(
model,
X,
y,
cv=cv,
return_estimator=True,
n_jobs=2,
)
coefs = pd.DataFrame(
[est[-1].regressor_.coef_ for est in cv_model["estimator"]], columns=feature_names
)
plt.ylabel("Age coefficient")
plt.xlabel("Experience coefficient")
plt.grid(True)
plt.xlim(-0.4, 0.5)
plt.ylim(-0.4, 0.5)
plt.scatter(coefs["AGE"], coefs["EXPERIENCE"])
_ = plt.title("Co-variations of coefficients for AGE and EXPERIENCE across folds")
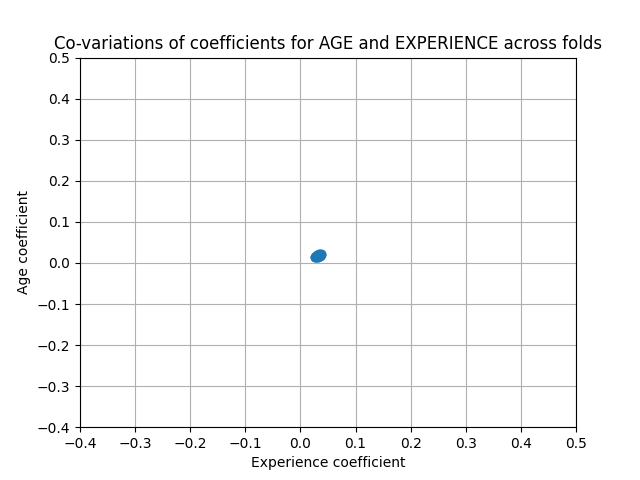
线性模型与稀疏系数#
另一种考虑数据集中相关变量的可能性是估计稀疏系数。在某种程度上,我们在之前的岭回归估计中手动删除AGE列时已经这样做了。
套索模型(参见 Lasso 用户指南部分)估计稀疏系数。LassoCV
应用交叉验证来确定哪个正则化参数( alpha
)值最适合模型估计。
from sklearn.linear_model import LassoCV
alphas = np.logspace(-10, 10, 21) # alpha values to be chosen from by cross-validation
model = make_pipeline(
preprocessor,
TransformedTargetRegressor(
regressor=LassoCV(alphas=alphas, max_iter=100_000),
func=np.log10,
inverse_func=sp.special.exp10,
),
)
_ = model.fit(X_train, y_train)
首先,我们验证选择了哪个
model[-1].regressor_.alpha_
np.float64(0.001)
然后我们检查预测的质量。
mae_train = median_absolute_error(y_train, model.predict(X_train))
y_pred = model.predict(X_test)
mae_test = median_absolute_error(y_test, y_pred)
scores = {
"MedAE on training set": f"{mae_train:.2f} $/hour",
"MedAE on testing set": f"{mae_test:.2f} $/hour",
}
_, ax = plt.subplots(figsize=(6, 6))
display = PredictionErrorDisplay.from_predictions(
y_test, y_pred, kind="actual_vs_predicted", ax=ax, scatter_kwargs={"alpha": 0.5}
)
ax.set_title("Lasso model, optimum regularization")
for name, score in scores.items():
ax.plot([], [], " ", label=f"{name}: {score}")
ax.legend(loc="upper left")
plt.tight_layout()
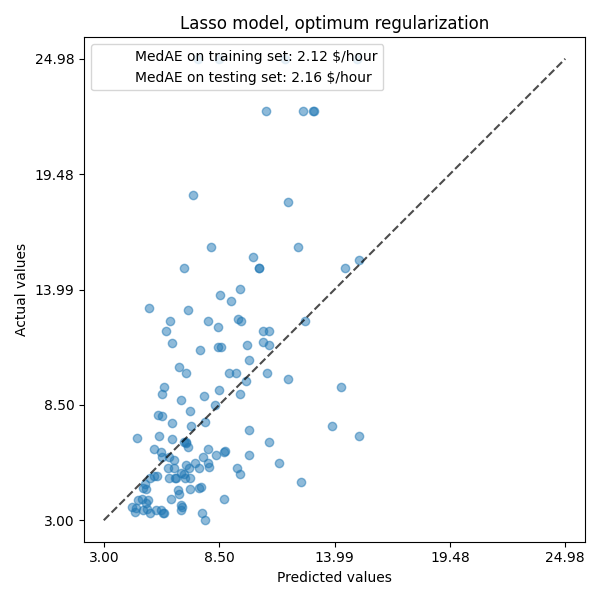
对于我们的数据集,模型的预测能力仍然不强。
coefs = pd.DataFrame(
model[-1].regressor_.coef_,
columns=["Coefficients importance"],
index=feature_names,
)
coefs.plot(kind="barh", figsize=(9, 7))
plt.title("Lasso model, optimum regularization, normalized variables")
plt.axvline(x=0, color=".5")
plt.subplots_adjust(left=0.3)
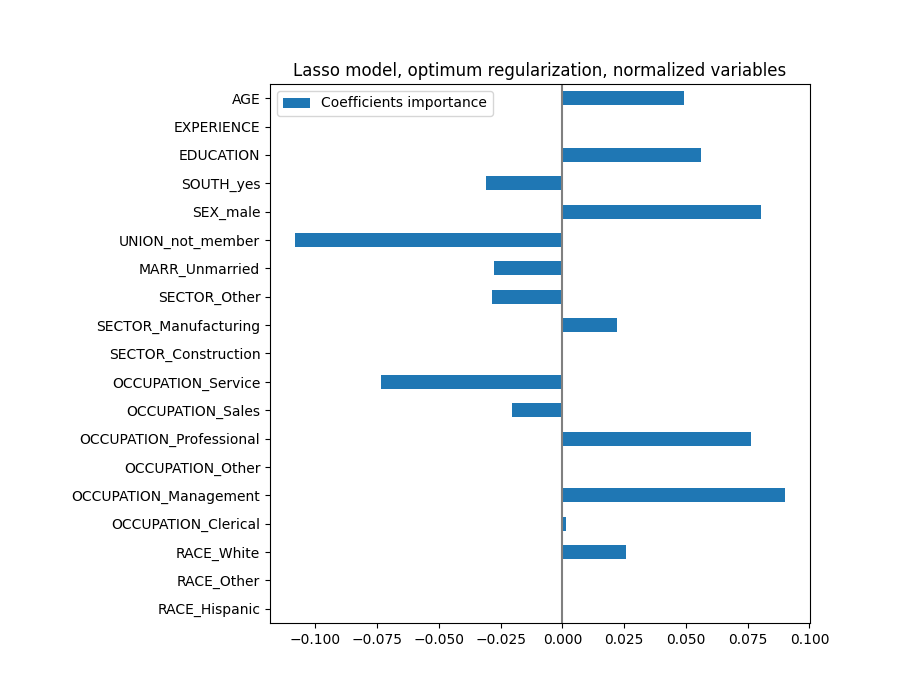
一个套索模型识别出年龄和经验之间的相关性,并为了预测的目的抑制其中一个。
请记住,被删除的系数本身可能仍然与结果相关:模型选择抑制它们是因为它们在其他特征之上带来的附加信息很少或没有。此外,对于相关特征,这种选择是不稳定的,应谨慎解释。
确实,我们可以检查不同折叠中系数的变化情况。
cv_model = cross_validate(
model,
X,
y,
cv=cv,
return_estimator=True,
n_jobs=2,
)
coefs = pd.DataFrame(
[est[-1].regressor_.coef_ for est in cv_model["estimator"]], columns=feature_names
)
plt.figure(figsize=(9, 7))
sns.stripplot(data=coefs, orient="h", palette="dark:k", alpha=0.5)
sns.boxplot(data=coefs, orient="h", color="cyan", saturation=0.5, whis=100)
plt.axvline(x=0, color=".5")
plt.title("Coefficient variability")
plt.subplots_adjust(left=0.3)
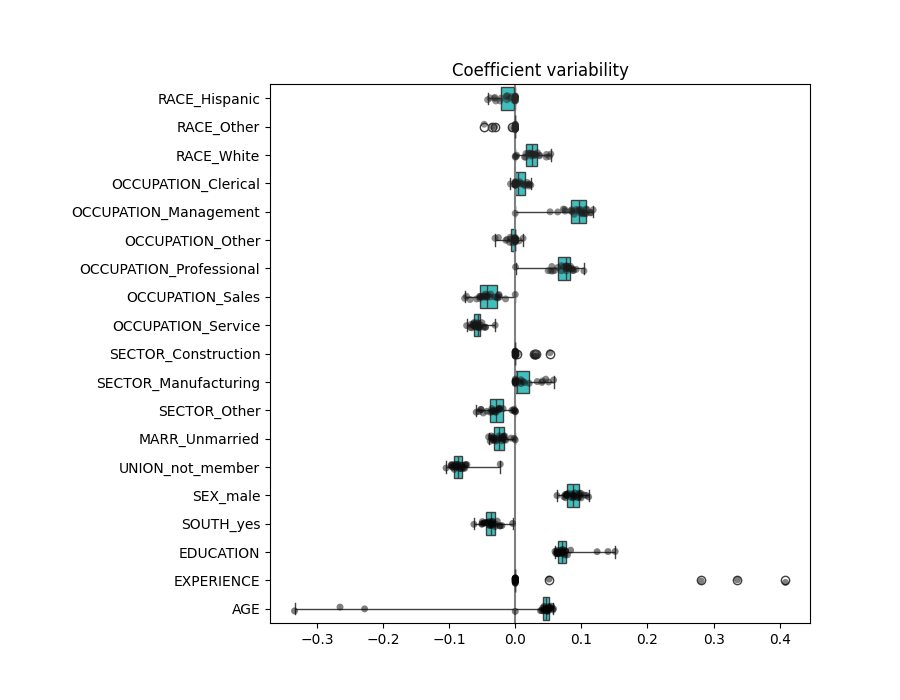
我们观察到,AGE 和 EXPERIENCE 系数在不同的折叠中变化很大。
错误的因果解释#
政策制定者可能希望了解教育对工资的影响,以评估某项旨在吸引人们接受更多教育的政策是否具有经济意义。虽然机器学习模型在衡量统计关联方面表现出色,但它们通常无法推断因果效应。
可能会很诱人地想要通过我们上一个模型(或任何模型)的教育系数来判断其对工资的影响,并得出它捕捉到了标准化教育变量变化对工资的真实影响的结论。
不幸的是,可能存在未观察到的混杂变量,这些变量会使该系数膨胀或缩小。混杂变量是指同时影响教育(EDUCATION)和工资(WAGE)的变量。一个例子是能力(ability)。可以假设,更有能力的人更有可能追求教育,同时在任何教育水平上更有可能获得更高的小时工资。在这种情况下,能力对教育系数产生了正向的“遗漏变量偏差(Omitted Variable Bias,OVB)”,从而夸大了教育对工资的影响。
请参阅 机器学习在推断因果效应方面的失败 了解一个模拟的能力OVB案例。
经验教训#
系数必须缩放到相同的度量单位才能获取特征重要性。用特征的标准差进行缩放是一个有用的替代方法。
当存在混杂效应时,解释因果关系是困难的。如果两个变量之间的关系也受到未观察到的因素的影响,我们在得出因果关系结论时应谨慎。
多变量线性模型中的系数表示给定特征与目标之间的依赖关系, 条件是 其他特征不变。
相关特征会在线性模型的系数中引发不稳定性,并且它们的影响难以很好地区分开来。
不同的线性模型对特征相关性的响应不同,系数可能会显著地彼此不同。
检查交叉验证循环各折中的系数可以了解它们的稳定性。
系数不太可能具有任何因果意义。它们往往会受到未观察到的混杂因素的偏倚。
检查工具不一定能提供对真实数据生成过程的见解。
Total running time of the script: (0 minutes 11.789 seconds)
Related examples