Note
Go to the end to download the full example code. or to run this example in your browser via Binder
绘制个体和投票回归预测#
投票回归器是一种集成元估计器,它拟合多个基回归器,每个基回归器都在整个数据集上进行训练。然后,它对各个预测结果进行平均,以形成最终预测。
我们将使用三种不同的回归器来预测数据:
GradientBoostingRegressor
、
RandomForestRegressor
和
LinearRegression
)。
然后,上述三个回归器将用于
VotingRegressor
。
最后,我们将绘制所有模型的预测结果以进行比较。
我们将使用糖尿病数据集,该数据集包含从一组糖尿病患者中收集的10个特征。目标是一年后基线的疾病进展的定量测量。
import matplotlib.pyplot as plt
from sklearn.datasets import load_diabetes
from sklearn.ensemble import (
GradientBoostingRegressor,
RandomForestRegressor,
VotingRegressor,
)
from sklearn.linear_model import LinearRegression
训练分类器#
首先,我们将加载糖尿病数据集,并初始化一个梯度提升回归器、一个随机森林回归器和一个线性回归。接下来,我们将使用这三个回归器来构建投票回归器:
X, y = load_diabetes(return_X_y=True)
# 训练分类器
reg1 = GradientBoostingRegressor(random_state=1)
reg2 = RandomForestRegressor(random_state=1)
reg3 = LinearRegression()
reg1.fit(X, y)
reg2.fit(X, y)
reg3.fit(X, y)
ereg = VotingRegressor([("gb", reg1), ("rf", reg2), ("lr", reg3)])
ereg.fit(X, y)
进行预测#
现在我们将使用每个回归器进行前20次预测。
xt = X[:20]
pred1 = reg1.predict(xt)
pred2 = reg2.predict(xt)
pred3 = reg3.predict(xt)
pred4 = ereg.predict(xt)
绘制结果#
最后,我们将可视化20个预测结果。红色星星表示由 VotingRegressor
做出的平均预测。
plt.figure()
plt.plot(pred1, "gd", label="GradientBoostingRegressor")
plt.plot(pred2, "b^", label="RandomForestRegressor")
plt.plot(pred3, "ys", label="LinearRegression")
plt.plot(pred4, "r*", ms=10, label="VotingRegressor")
plt.tick_params(axis="x", which="both", bottom=False, top=False, labelbottom=False)
plt.ylabel("predicted")
plt.xlabel("training samples")
plt.legend(loc="best")
plt.title("Regressor predictions and their average")
plt.show()
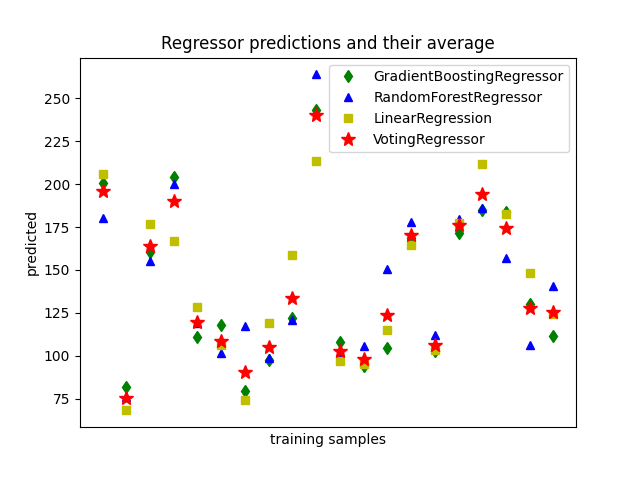
Total running time of the script: (0 minutes 1.374 seconds)
Related examples