Note
Go to the end to download the full example code. or to run this example in your browser via Binder
使用鸢尾花数据集的PCA示例#
主成分分析应用于鸢尾花数据集。
有关此数据集的更多信息,请参见 这里 。
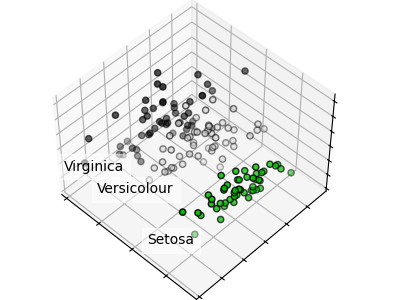
# 代码来源:Gaël Varoquaux
# SPDX许可证标识符:BSD-3-Clause
import matplotlib.pyplot as plt
# 未使用但需要导入以使用低于3.2版本的matplotlib进行3D投影
import mpl_toolkits.mplot3d # noqa: F401
import numpy as np
from sklearn import datasets, decomposition
np.random.seed(5)
iris = datasets.load_iris()
X = iris.data
y = iris.target
fig = plt.figure(1, figsize=(4, 3))
plt.clf()
ax = fig.add_subplot(111, projection="3d", elev=48, azim=134)
ax.set_position([0, 0, 0.95, 1])
plt.cla()
pca = decomposition.PCA(n_components=3)
pca.fit(X)
X = pca.transform(X)
for name, label in [("Setosa", 0), ("Versicolour", 1), ("Virginica", 2)]:
ax.text3D(
X[y == label, 0].mean(),
X[y == label, 1].mean() + 1.5,
X[y == label, 2].mean(),
name,
horizontalalignment="center",
bbox=dict(alpha=0.5, edgecolor="w", facecolor="w"),
)
# 重新排序标签以使颜色与聚类结果匹配
y = np.choose(y, [1, 2, 0]).astype(float)
ax.scatter(X[:, 0], X[:, 1], X[:, 2], c=y, cmap=plt.cm.nipy_spectral, edgecolor="k")
ax.xaxis.set_ticklabels([])
ax.yaxis.set_ticklabels([])
ax.zaxis.set_ticklabels([])
plt.show()
Total running time of the script: (0 minutes 0.038 seconds)
Related examples