Note
Go to the end to download the full example code. or to run this example in your browser via Binder
scikit-learn 1.5 版本发布亮点#
我们很高兴地宣布发布 scikit-learn 1.5!此次更新包含了许多错误修复和改进,以及一些关键的新功能。以下是本次发布的亮点。 有关所有更改的详尽列表 ,请参阅 发布说明 。
要安装最新版本(使用 pip):
pip install --upgrade scikit-learn
或使用 conda:
conda install -c conda-forge scikit-learn
固定阈值分类器:设置二元分类器的决策阈值#
scikit-learn的所有二元分类器都使用固定的0.5决策阈值,将概率估计(即 predict_proba
的输出)转换为类别预测。然而,对于给定的问题,0.5几乎从来不是理想的阈值。FixedThresholdClassifier
允许包装任何二元分类器并设置自定义决策阈值。
from sklearn.datasets import make_classification
from sklearn.model_selection import train_test_split
from sklearn.linear_model import LogisticRegression
from sklearn.metrics import ConfusionMatrixDisplay
X, y = make_classification(n_samples=10_000, weights=[0.9, 0.1], random_state=0)
X_train, X_test, y_train, y_test = train_test_split(X, y, random_state=0)
classifier_05 = LogisticRegression(C=1e6, random_state=0).fit(X_train, y_train)
_ = ConfusionMatrixDisplay.from_estimator(classifier_05, X_test, y_test)
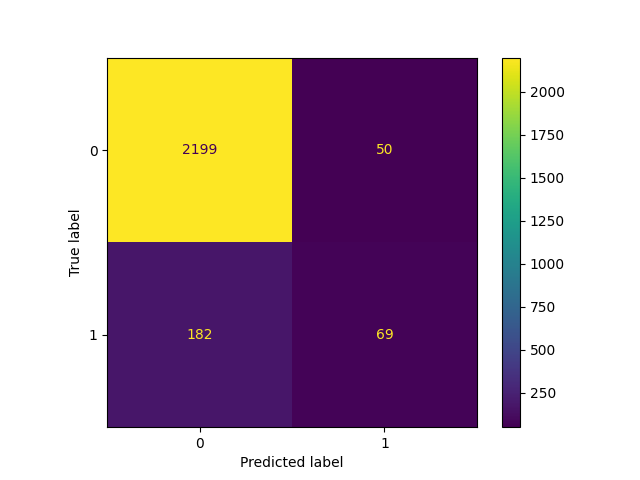
降低阈值,即允许更多样本被分类为正类,会增加真正例的数量,但代价是更多的假正例(这在ROC曲线的凹性中是众所周知的)。
from sklearn.model_selection import FixedThresholdClassifier
classifier_01 = FixedThresholdClassifier(classifier_05, threshold=0.1)
classifier_01.fit(X_train, y_train)
_ = ConfusionMatrixDisplay.from_estimator(classifier_01, X_test, y_test)
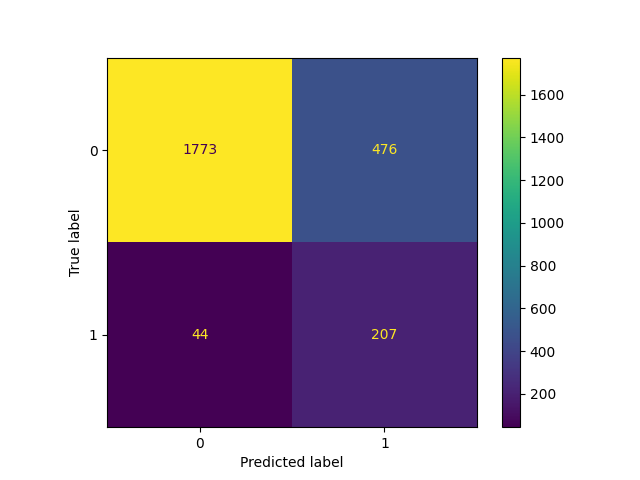
调优阈值分类器CV:调优二元分类器的决策阈值#
可以使用 TunedThresholdClassifierCV
调优二元分类器的决策阈值,以优化给定的指标。
它特别适用于在模型要部署到特定应用场景时找到最佳决策阈值,在这种场景中,我们可以为真正例、真负例、假正例和假负例分配不同的收益或成本。
让我们通过考虑一个任意的案例来说明这一点:
每个真正例获得1单位的利润,例如欧元、健康生活年限等;
真负例不获得也不损失任何东西;
每个假负例损失2单位;
每个假正例损失0.1单位。
我们的指标量化了每个样本的平均利润,其定义如下Python函数:
from sklearn.metrics import confusion_matrix
def custom_score(y_observed, y_pred):
tn, fp, fn, tp = confusion_matrix(y_observed, y_pred, normalize="all").ravel()
return tp - 2 * fn - 0.1 * fp
print("Untuned decision threshold: 0.5")
print(f"Custom score: {custom_score(y_test, classifier_05.predict(X_test)):.2f}")
Untuned decision threshold: 0.5
Custom score: -0.12
有趣的是,观察到每次预测的平均收益为负,这意味着该决策系统平均在亏损。
调整阈值以优化此自定义指标会得到一个较小的阈值,从而允许更多样本被分类为正类。结果是,每次预测的平均收益提高。
from sklearn.model_selection import TunedThresholdClassifierCV
from sklearn.metrics import make_scorer
custom_scorer = make_scorer(
custom_score, response_method="predict", greater_is_better=True
)
tuned_classifier = TunedThresholdClassifierCV(
classifier_05, cv=5, scoring=custom_scorer
).fit(X, y)
print(f"Tuned decision threshold: {tuned_classifier.best_threshold_:.3f}")
print(f"Custom score: {custom_score(y_test, tuned_classifier.predict(X_test)):.2f}")
Tuned decision threshold: 0.071
Custom score: 0.04
我们观察到,调整决策阈值可以将一个平均上会造成损失的基于机器学习的系统转变为一个有益的系统。
在实际操作中,定义一个有意义的特定应用指标可能涉及到使错误预测的成本和正确预测的收益依赖于每个数据点特有的辅助元数据,例如在欺诈检测系统中交易金额。
为实现这一目标,TunedThresholdClassifierCV
利用元数据路由支持 (元数据路由用户指南 ) 允许优化复杂的业务指标,详见 成本敏感学习的决策阈值后调优 。
PCA中的性能改进#
PCA
有一个新的求解器 "covariance_eigh"
,对于具有大量数据点和少量特征的数据集,该求解器比其他求解器快一个数量级,并且更节省内存。
from sklearn.datasets import make_low_rank_matrix
from sklearn.decomposition import PCA
X = make_low_rank_matrix(
n_samples=10_000, n_features=100, tail_strength=0.1, random_state=0
)
pca = PCA(n_components=10, svd_solver="covariance_eigh").fit(X)
print(f"Explained variance: {pca.explained_variance_ratio_.sum():.2f}")
Explained variance: 0.88
新的求解器也接受稀疏输入数据:
Explained variance: 0.13
"full"
求解器也得到了改进,使用更少的内存并允许更快的转换。默认的 svd_solver="auto"
选项利用了新的求解器,现在能够为稀疏数据集选择合适的求解器。
与大多数其他PCA求解器类似,如果通过启用对 Array API 的实验性支持,将输入数据作为PyTorch或CuPy数组传递,则新的 "covariance_eigh"
求解器可以利用GPU计算。
ColumnTransformer 是可下标的#
现在可以通过名称索引直接访问 ColumnTransformer
的转换器。
import numpy as np
from sklearn.compose import ColumnTransformer
from sklearn.preprocessing import StandardScaler, OneHotEncoder
X = np.array([[0, 1, 2], [3, 4, 5]])
column_transformer = ColumnTransformer(
[("std_scaler", StandardScaler(), [0]), ("one_hot", OneHotEncoder(), [1, 2])]
)
column_transformer.fit(X)
print(column_transformer["std_scaler"])
print(column_transformer["one_hot"])
StandardScaler()
OneHotEncoder()
自定义SimpleImputer的填补策略#
SimpleImputer
现在支持使用自定义策略进行填补,
可以使用一个可调用对象从列向量的非缺失值中计算出一个标量值。
from sklearn.impute import SimpleImputer
X = np.array(
[
[-1.1, 1.1, 1.1],
[3.9, -1.2, np.nan],
[np.nan, 1.3, np.nan],
[-0.1, -1.4, -1.4],
[-4.9, 1.5, -1.5],
[np.nan, 1.6, 1.6],
]
)
def smallest_abs(arr):
"""返回一维数组的最小绝对值。"""
return np.min(np.abs(arr))
imputer = SimpleImputer(strategy=smallest_abs)
imputer.fit_transform(X)
array([[-1.1, 1.1, 1.1],
[ 3.9, -1.2, 1.1],
[ 0.1, 1.3, 1.1],
[-0.1, -1.4, -1.4],
[-4.9, 1.5, -1.5],
[ 0.1, 1.6, 1.6]])
成对距离与非数值数组#
pairwise_distances
现在可以使用可调用的度量来计算非数值数组之间的距离。
from sklearn.metrics import pairwise_distances
X = ["cat", "dog"]
Y = ["cat", "fox"]
def levenshtein_distance(x, y):
"""返回两个字符串之间的Levenshtein距离。"""
if x == "" or y == "":
return max(len(x), len(y))
if x[0] == y[0]:
return levenshtein_distance(x[1:], y[1:])
return 1 + min(
levenshtein_distance(x[1:], y),
levenshtein_distance(x, y[1:]),
levenshtein_distance(x[1:], y[1:]),
)
pairwise_distances(X, Y, metric=levenshtein_distance)
array([[0., 3.],
[3., 2.]])
Total running time of the script: (0 minutes 0.734 seconds)
Related examples