Note
Go to the end to download the full example code. or to run this example in your browser via Binder
使用贝叶斯岭回归进行曲线拟合#
计算正弦曲线的贝叶斯岭回归。
有关回归器的更多信息,请参见 贝叶斯岭回归 。
通常,在使用贝叶斯岭回归通过多项式拟合曲线时,正则化参数(alpha, lambda)的初始值选择可能很重要。这是因为正则化参数是通过依赖初始值的迭代过程确定的。
在此示例中,使用不同的初始值对来通过多项式逼近正弦曲线。
当从默认值(alpha_init = 1.90, lambda_init = 1.)开始时,结果曲线的偏差较大,方差较小。因此,lambda_init 应相对较小(1.e-3),以减少偏差。
此外,通过评估这些模型的对数边际似然(L),我们可以确定哪个模型更好。可以得出结论,L 较大的模型更有可能。
# Author: Yoshihiro Uchida <nimbus1after2a1sun7shower@gmail.com>
生成带噪声的正弦数据#
import numpy as np
def func(x):
return np.sin(2 * np.pi * x)
size = 25
rng = np.random.RandomState(1234)
x_train = rng.uniform(0.0, 1.0, size)
y_train = func(x_train) + rng.normal(scale=0.1, size=size)
x_test = np.linspace(0.0, 1.0, 100)
按三次多项式拟合#
from sklearn.linear_model import BayesianRidge
n_order = 3
X_train = np.vander(x_train, n_order + 1, increasing=True)
X_test = np.vander(x_test, n_order + 1, increasing=True)
reg = BayesianRidge(tol=1e-6, fit_intercept=False, compute_score=True)
绘制真实和预测曲线与对数边际似然 (L)#
import matplotlib.pyplot as plt
fig, axes = plt.subplots(1, 2, figsize=(8, 4))
for i, ax in enumerate(axes):
# 不同初始值对的贝叶斯岭回归
if i == 0:
init = [1 / np.var(y_train), 1.0] # Default values
elif i == 1:
init = [1.0, 1e-3]
reg.set_params(alpha_init=init[0], lambda_init=init[1])
reg.fit(X_train, y_train)
ymean, ystd = reg.predict(X_test, return_std=True)
ax.plot(x_test, func(x_test), color="blue", label="sin($2\\pi x$)")
ax.scatter(x_train, y_train, s=50, alpha=0.5, label="observation")
ax.plot(x_test, ymean, color="red", label="predict mean")
ax.fill_between(
x_test, ymean - ystd, ymean + ystd, color="pink", alpha=0.5, label="predict std"
)
ax.set_ylim(-1.3, 1.3)
ax.legend()
title = "$\\alpha$_init$={:.2f},\\ \\lambda$_init$={}$".format(init[0], init[1])
if i == 0:
title += " (Default)"
ax.set_title(title, fontsize=12)
text = "$\\alpha={:.1f}$\n$\\lambda={:.3f}$\n$L={:.1f}$".format(
reg.alpha_, reg.lambda_, reg.scores_[-1]
)
ax.text(0.05, -1.0, text, fontsize=12)
plt.tight_layout()
plt.show()
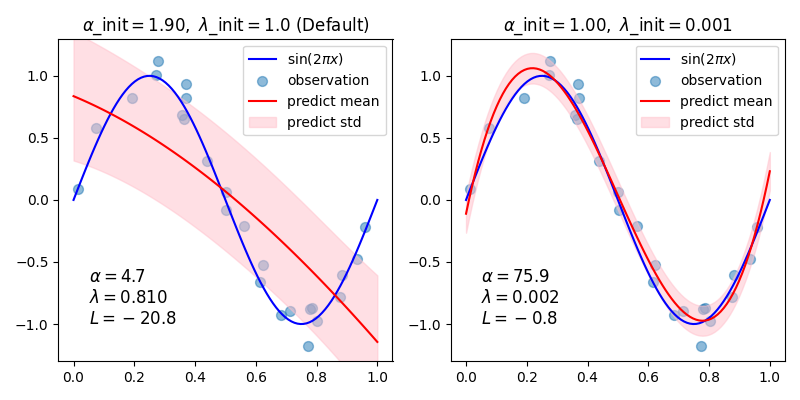
Total running time of the script: (0 minutes 0.133 seconds)
Related examples